Luận án Nonlinear distortions and countermeasures for performance improvements in contemporary radio communication systems
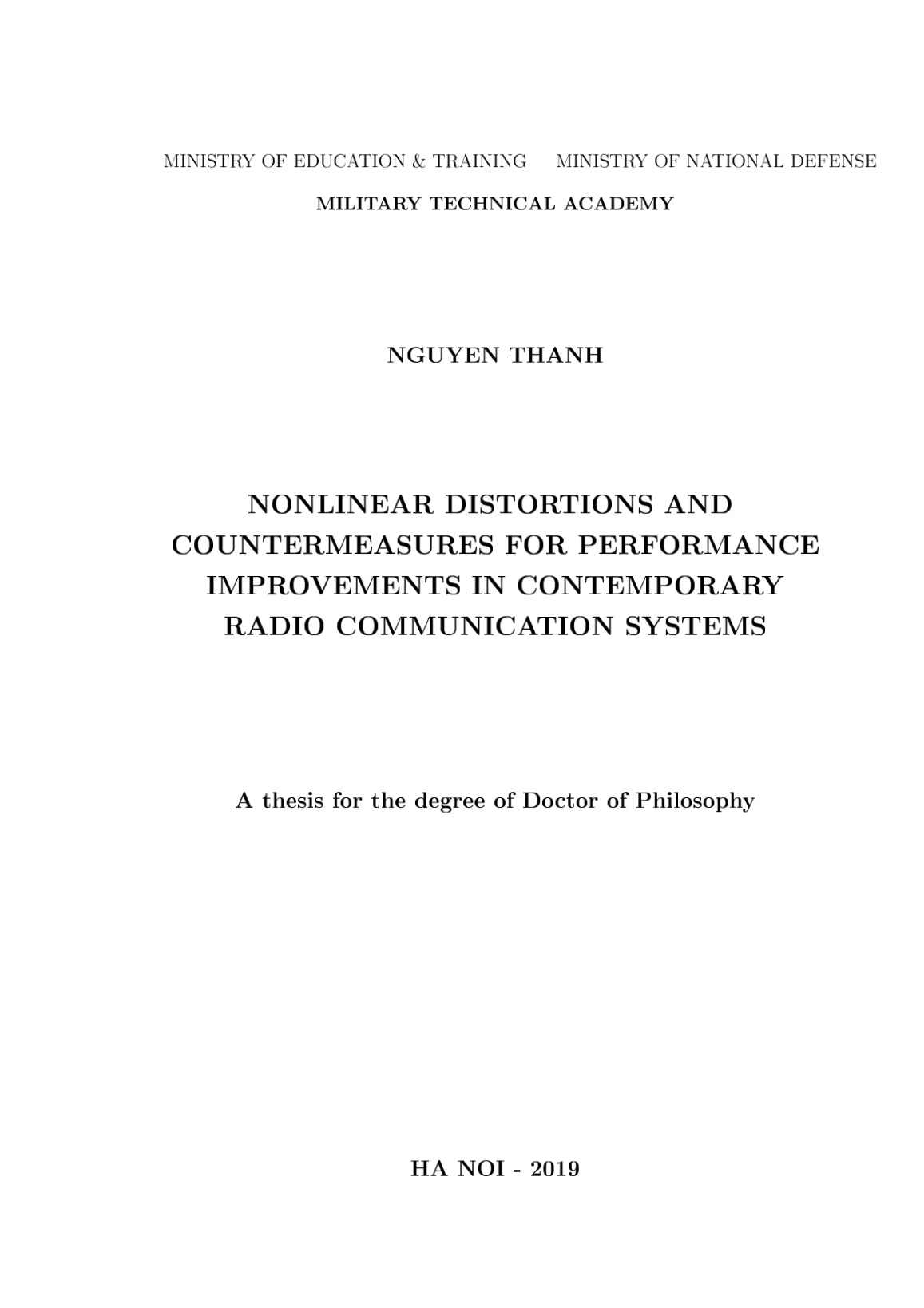
Trang 1
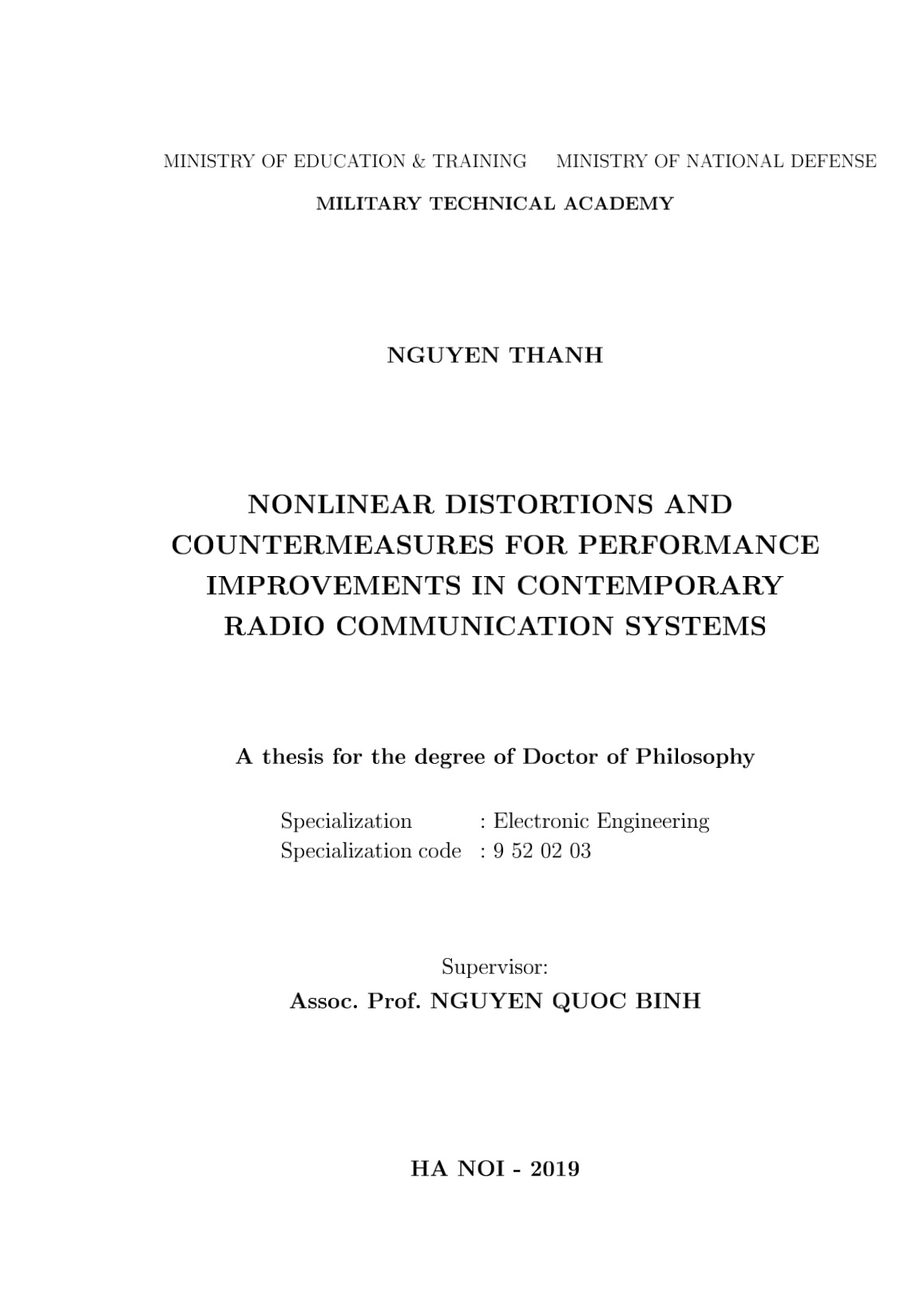
Trang 2
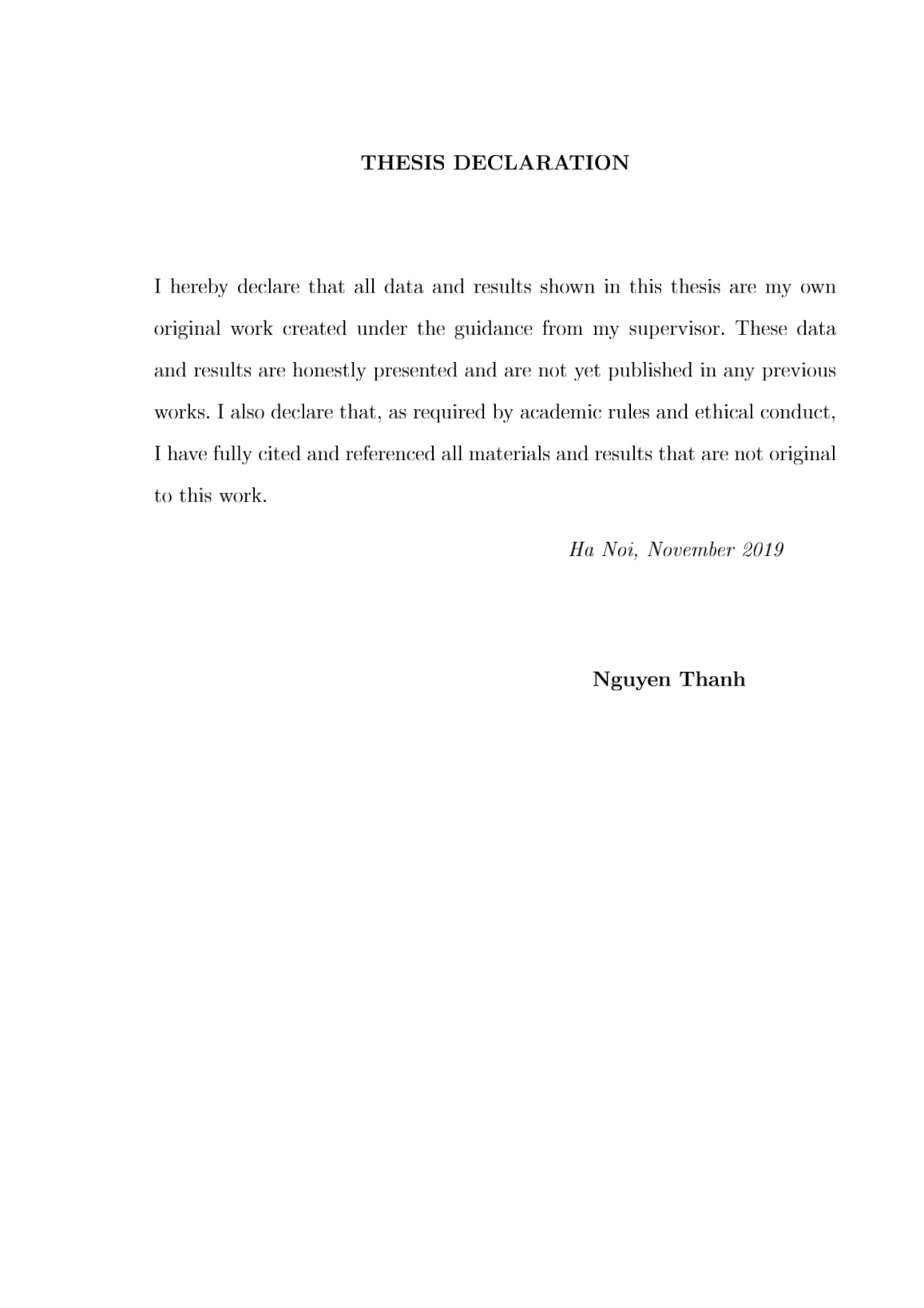
Trang 3
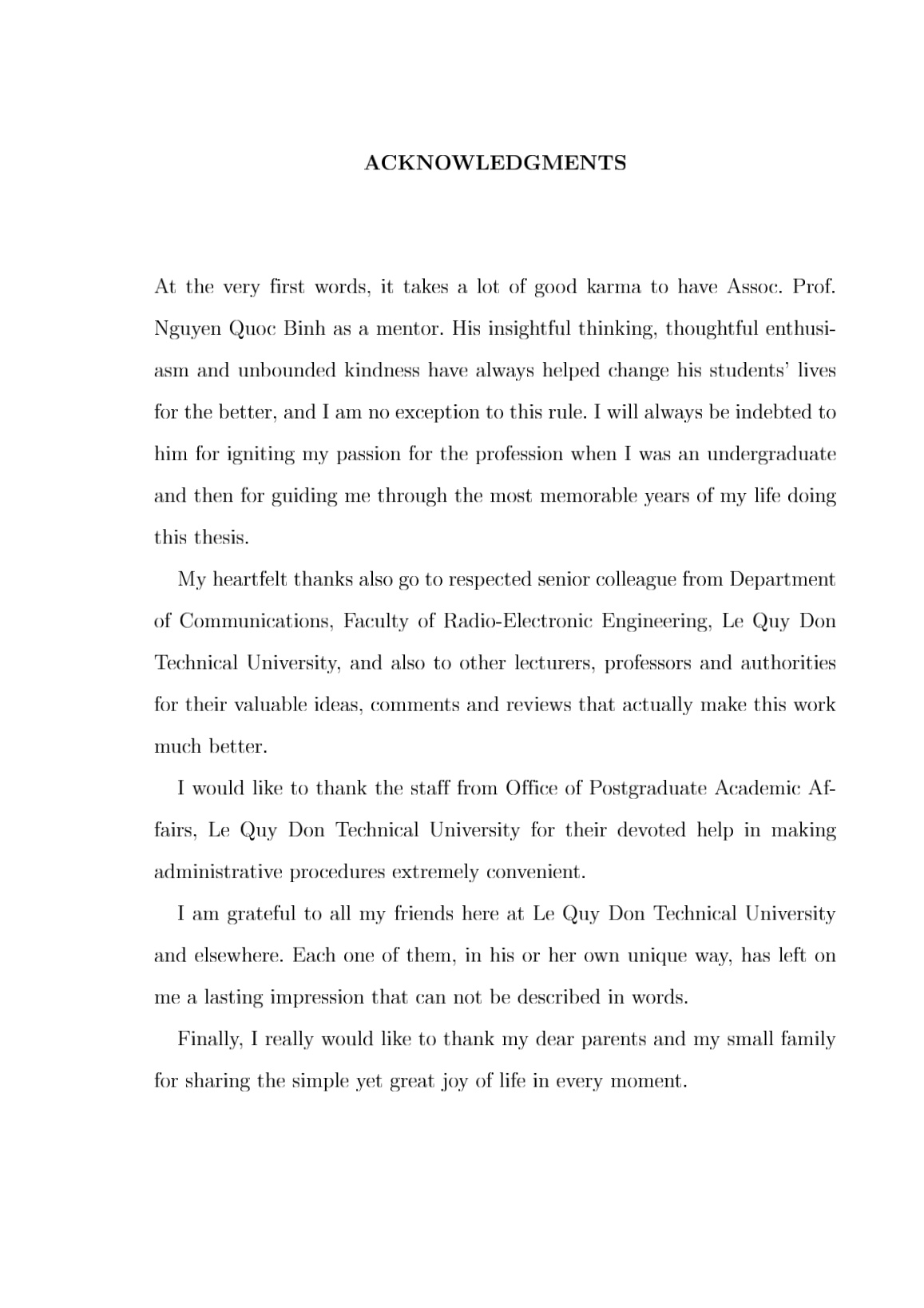
Trang 4
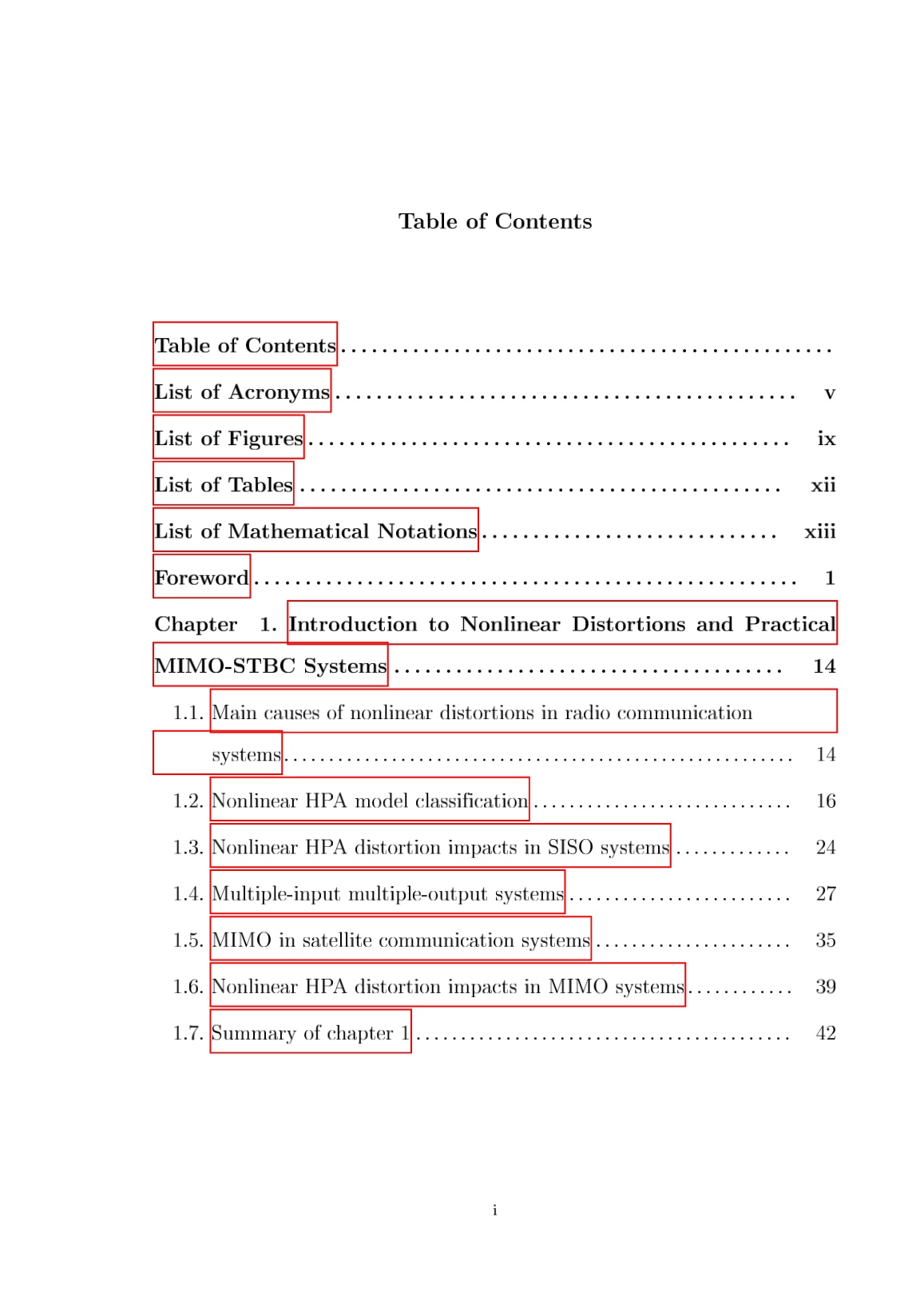
Trang 5
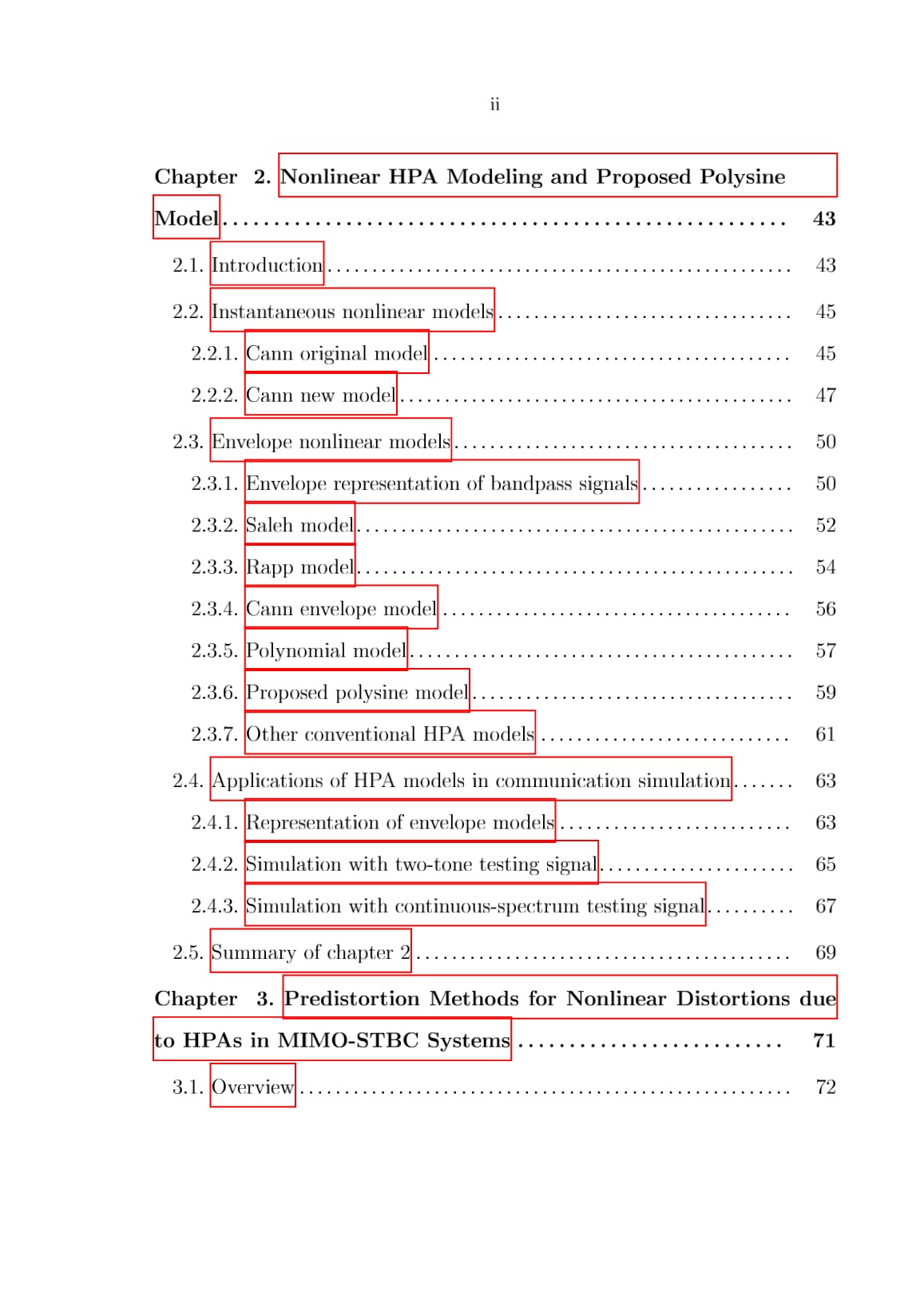
Trang 6
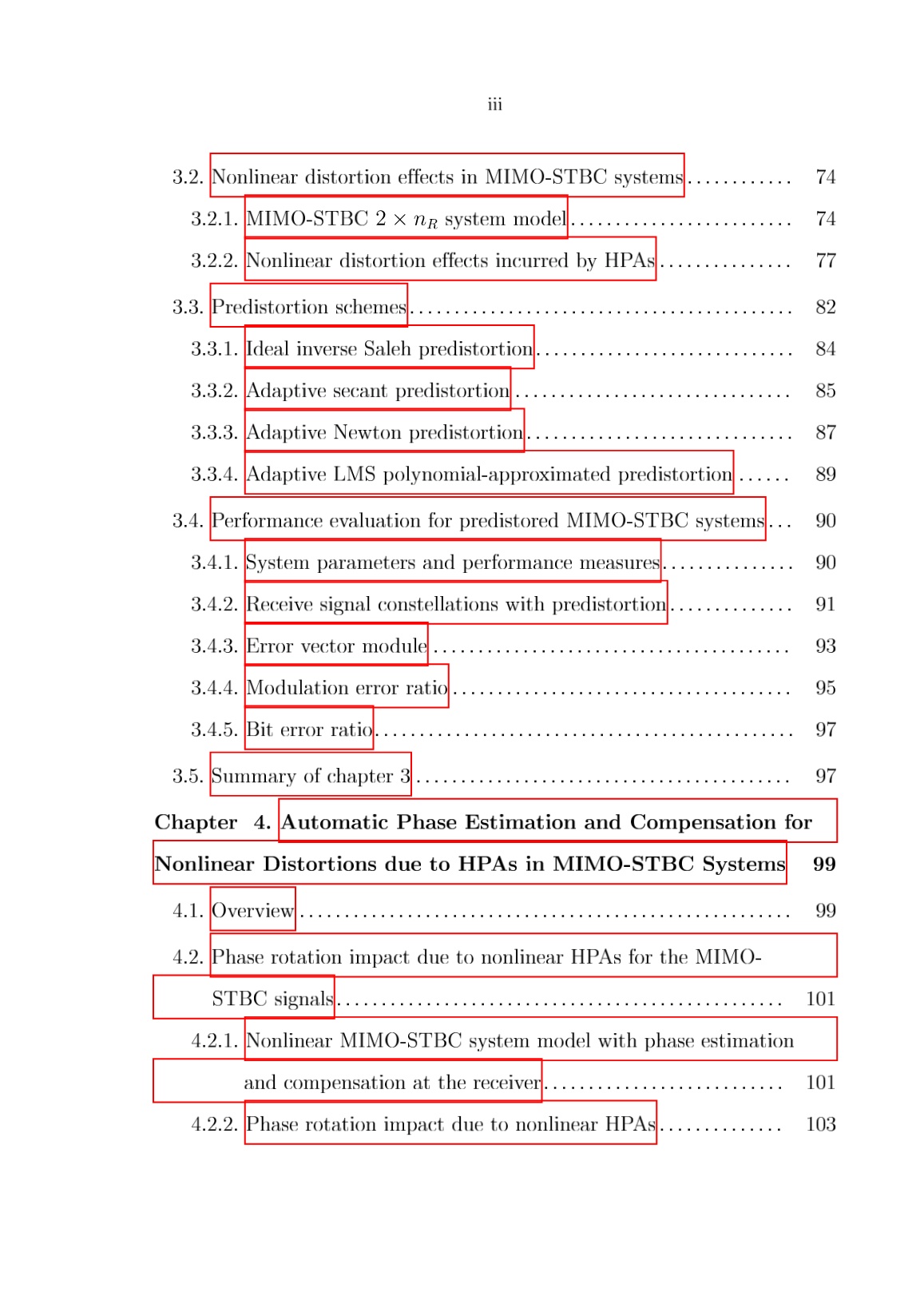
Trang 7
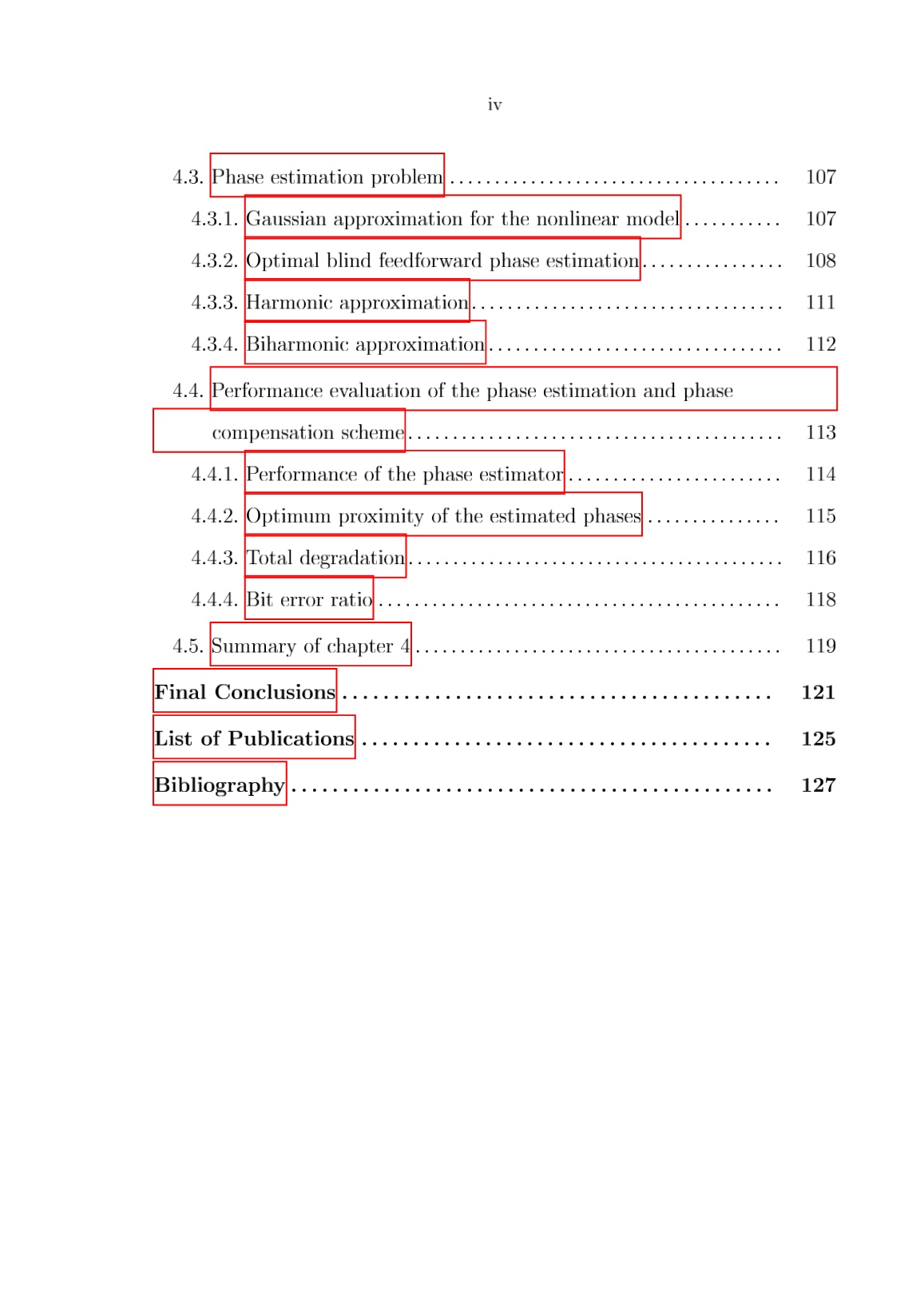
Trang 8
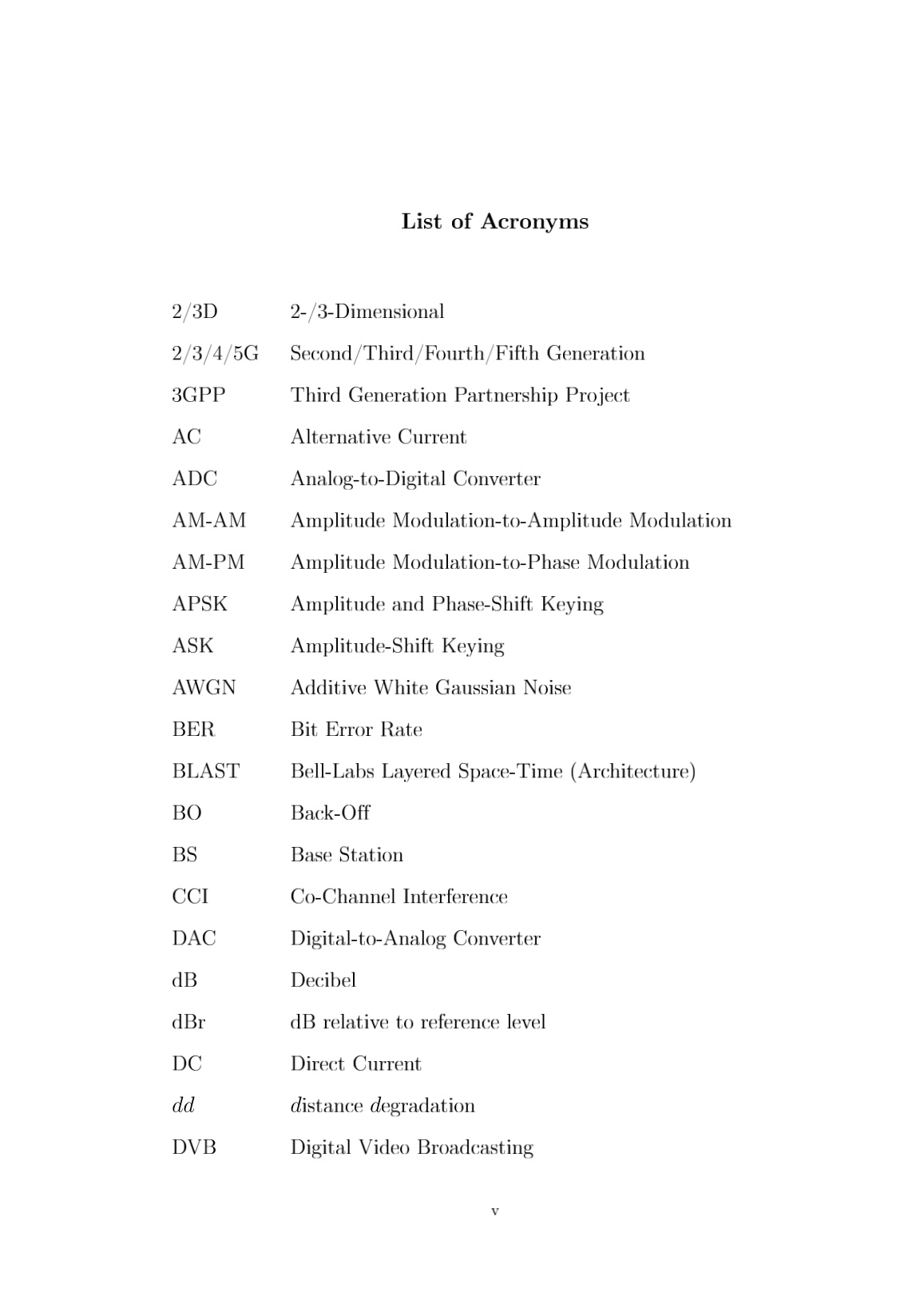
Trang 9
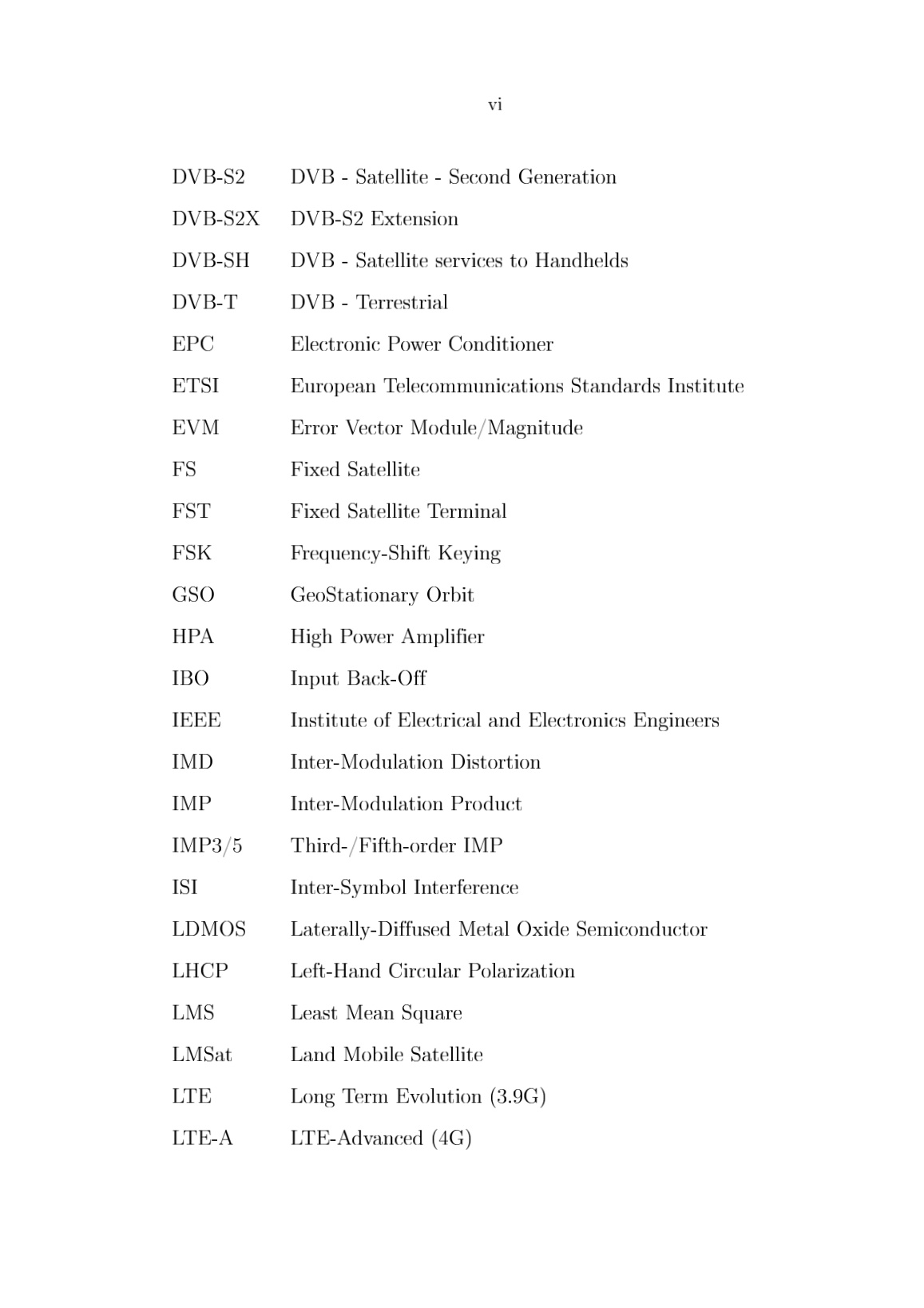
Trang 10
Tải về để xem bản đầy đủ
Bạn đang xem 10 trang mẫu của tài liệu "Luận án Nonlinear distortions and countermeasures for performance improvements in contemporary radio communication systems", để tải tài liệu gốc về máy hãy click vào nút Download ở trên.
Tóm tắt nội dung tài liệu: Luận án Nonlinear distortions and countermeasures for performance improvements in contemporary radio communication systems
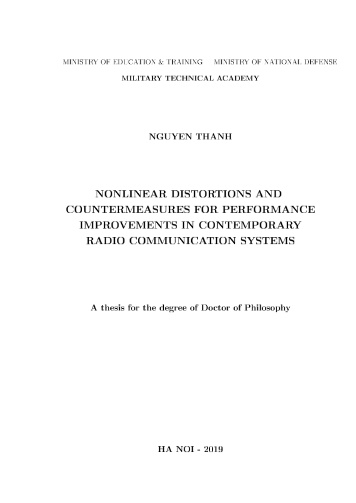
of useful signal energy converted to heat) reduces the amplification efficiency. However, the input dynamic range and the overheating endurance capability of SSPA are much different (inferior) compared to that of TWTA [100]. Thus, it is not easy to observe the turndown region after saturation; SSPA might be destroyed before approaching saturation by overheating or input overvoltage. 54 tortion. This form is described by I(r) = er 1 + fr2 , (2.9) Q(r) = gr3 (1 + hr2)2 , (2.10) where parameters e, f, g, h form the curve shapes. Also noting that (2.9) and (2.10) are both odd functions. 2.3.3. Rapp model In 1991, when studying the impacts of nonlinear HPAs in digital audio broadcasting systems, Rapp proposed an envelope model with variable knee sharpness for SSPAs as follows [82] Fa(r) = gr[ 1 + ( gr A os )2s]1/2s , (2.11) where r and Fa(r) correspondingly are the input and output amplitudes, g is the small-signal (linear) gain, Aos is the output saturation level, and s is the curve's sharpness controlling the transition from the linear region to the limiting region. It is noteworthy that this model assumed zero AM- PM conversion and by changing the sharpness parameter s, the AM-AM characteristic could have any curvature. On the other hand, (2.11) is an odd function (Saleh condition) only for integer s. Several examples of (2.11) with different knee sharpnesses s are illustrated in Figure 2.6 with normalized linear gain and output saturation level, i.e. g = 1, Aos = 1 [V]. In addition to this, the normalized characteristic curve of the ideal limiter (the upper most curve in Figure 2.4) is included for reference purpose. Noting again, this is an upper bound for any real-world amplifier 55 0 0.25 0.5 0.75 1 1.25 1.5 1.75 2 0 0.2 0.4 0.6 0.8 1 1.2 Input voltage [V] O ut pu t v ol ta ge [V ] Ideal limiter Rapp, s = 1 Rapp, s = 1.4 Rapp, s = 3 Rapp, s = ∞ Figure 2.6: AM-AM functions of the Rapp model with different sharpnesses. (with an asymptotic exception of ideal predistorter-amplifier combination [32, 100]). Incidentally, this model resembles to the instantaneous model (2.1) except- ing the absence of modulus operator (|.|) in the denominator. Thus, it seems to avoid the problem of (2.1) for the suitability of IMPs resulted by simu- lation, but this is not the case. The Rapp's model has been widely used for roughly a quarter of century without any considerations for its reasonableness and also its suspicious results until the publication of Cann [18]. Thorough investigation leads to the conclusion that the problem of (2.1) in simulating IMPs only manifests with signals whose magnitude distributions concentrating around zero, such as the signal used in the two-tone test (that will be adequately investigated in subsection 2.4.2). For real-world signals like M -FSK,M -PSK,M -QAM,M -APSK, OFDM,. . . the Rapp's model behaves almost perfectly well. Therefore, resembling to the case of instantaneous mod- els, all envelope AM-AM models should ideally be odd and analytic 7 over the 7 The analyticity requirement for both instantaneous and envelope models could be intuitively explained as follows. To yield precise IMP results from time-domain (instantaneous or envelope) signals being passed through a nonlinear model, then information related to all frequency components (physically, being rates of change over time) of these waveform signals should be uninterruptedly transferred through the model. This means the model's transfer function should be smooth for all frequency components, or mathematically equivalent to being infinitely differentiable. When this condition is not satisfied, some frequency components will not be transferred through the model, directly affecting 56 expected amplitude range. An envelope model, which is asymmetric and is not analytic at zero, should be used with caution and only for signal waveforms that are sufficiently complex to have a widespread amplitude distribution with small possibility of zero amplitude. Thus, this problem, once having not been widely understood though being relatively important, is now clear. It is then clearer when considering the mutual effects of three factors: model's analyticity, data-approximation accuracy and signal's amplitude distribution in simulating IMPs. This is particularly discussed in section 2.4. 2.3.4. Cann envelope model Although originally developed as an instantaneous model, (2.2) can be used equally as an envelope model. In this case, it is not the right halves of curves in Figure 2.4 but those in Figure 2.1. This model should find broad applications, particularly for SSPAs since, like Rapp model, it has adjustable knee sharpness and does not turn down after saturation. But, unlike the Rapp model, it is analytic everywhere and therefore valid for any signal waveform. Moreover, if the phase conversion is significant, an AM-PM function, like Saleh's (2.8), could be included. Similar to the Rapp model (2.11), envelope model (2.2) could support any curvatures, especially in the region above s = 2.5, suitable for AM- AM characteristics of most SSPAs [32]. The approximations of the Cann new model (2.2) and Rapp model (2.11) to the real-world data are verified by curve fitting of these functions to the measured data from the L band Quasonix 10W amplifier [86]. The results are, for Rapp model: g = 29.4, Aos = 30 [V], s = 4.15, Squared Error Sum (SES) σ 2 e = 0.963; for Cann the IMP values estimated at the output. 57 0 0.2 0.4 0.6 0.8 1 1.2 1.4 1.6 0 5 10 15 20 25 30 35 Input [V] O ut pu t [V ] Data Cann (2.2) Rapp (2.11) Polynomial (2.12) Polynomial (2.13) Polysine (2.14) 1.15 1.2 1.25 1.3 28.5 29 29.5 0.65 0.7 0.75 0.8 20 21 22 Figure 2.7: AM-AM functions of the Cann, Rapp, polynomial, odd-order polynomial and polysine models fitted to the measured data. new model: g = 29.4, Aos = 30 [V], s = 8.9 and σ 2 e = 1.786. For this particular HPA, Rapp model is little better fitted than Cann model. Figure 2.7 illustrates these fittings with the inclusion of other approximated curves discussed next. 2.3.5. Polynomial model Considering the measured data in Figure 2.7, it is not difficult to recog- nized that there is a simple yet efficient method approaching the closed-form AM-AM function by approximation using polynomials. In this case, the com- plex envelope nonlinearity, F (.), can be represented by a complex polynomial power series of a finite order N such that [32]: y = F (x) = N∑ n=1 an|x|n−1x = N∑ n=1 anΨ P n [x], (2.12) 58 Table 2.1: Coefficients of the polynomial models (2.12), (2.13). a1 30.02 a2 a3 a4 a5 a6 a7 a8 a9Model (2.12) (2.13) -8.665 33.68 28.60 0 8.310 -40.19 12.39 0 0 -15.06 0 0 0 0 6.257 0 -0.872 where ΨPn [x] = |x|n−1x are the basis functions of the polynomial model, and an are the model's complex coefficients of order n, n = 1, 2, ..., N . Obviously, model (2.12) is not analytic at r = |x| = 0 by the existence of modulus operators (|.|). However, if even order coefficients a2n vanish, then for real-valued x(t), (2.12) turns into the odd-order polynomial model of the form y = N∑ n=1 a2n−1|x|2(n−1)x = N∑ n=1 a2n−1x 2n−1. (2.13) Model (2.13) is clearly analytic at r = 0 and is used as a counter example to model (2.12), showing that though having almost similar structure, they give quite different results. This is discussed in the section 2.4 below. The measured data of the L band Quasonix 10W amplifier [86] is then used to fit the polynomial models (2.12) and (2.13) with the same number of coeffi- cients N = 5. Figure 2.7 depicts the approximated characteristics with their corresponding parameters listed in Table 2.1. It is not difficult to show that at large enough order, polynomial models are better fitted to the real-world data than Rapp model (2.11) and Cann new model (2.2). Moreover, with the same N , odd-order polynomial of (2.13) is obviously smoother than full order one of (2.12) resulting in better fitting performance for the former. However, it is also worthy of noting that using too high order for polynomial fitting leads to the Runge phenomenon [101], 59 which also does increase the approximating errors. 2.3.6. Proposed polysine model It can be seen that the sine/cosine functions are distinctly better than polynomial ones in terms of both analyticity and smoothness. Thus, while remaining to be analytic, the former are better fitted to the real-world data than the latter. Based on this fact, we propose a nonlinear model of the form y = N∑ n=1 an sin(bnx), (2.14) where an and bn are respectively amplitude and phase coefficients. Intuitively, the introduction of bn allows (2.14) better adapting to the data variations, thus further improves the approximation performance. On the other hand, mathematically, it is not too difficult to recognize that polysine model is equivalent to odd-order polynomial model. Definitely, applying the Taylor expansion at x = 0, function sin(x) could be decomposed as [41] sin(x) = M∑ m=1 (−1)m−1 x 2m−1 (2m− 1)! +O ( x2M−1 ) , (2.15) where (k)! is the factorial of order k, O (x2M−1) is the order of approximation error. Then, (2.14) is recast as y = N∑ n=1 an sin(bnx) = N∑ n=1 an ( M∑ m=1 (−1)m−1 (bnx) 2m−1 (2m− 1)! +O ( x2M−1 )) = M∑ m=1 ( N∑ n=1 (−1)m−1an(bn) 2m−1 (2m− 1)! ) x2m−1 +O (x2M−1) = M∑ m=1 a′mx 2m−1 +O (x2M−1). (2.16) 60 This is of the form (2.13) plus approximation error polynomial of order 2M− 1, O (x2M−1). Thus, mathematically, odd-order polynomial model is equiva- lent to polysine model. However, technically, it is the quantity of O (x2M−1) that allows polysine model being always better fitted to data compared to odd-order polynomial model (2.13) and thus also being superior to full order polynomial (2.12) as analyzed above. Besides the analyticity, the require- ment of small approximation error in modeling becomes truly critical when the model's output is used to evaluate high-order harmonics and intermodu- lation products, which commonly are quantities much smaller than the funda- mental harmonics/components. Obviously, model with lower approximation error yields more precise results. Demonstrating examples in section 2.4 could clarify this fact. Table 2.2: Coefficients of the polysine model (2.14). 1 30.73 2 3 4 5n an bn -0.6586 -0.1061 1.045 5.312 12.91 0.00955 0.1859 18.61 8.107 Using the Matlab curve fitting tool, (2.14) is fixed to the AM-AM charac- teristic of the L band Quasonix 10W amplifier data in Figure 2.7 resulting in parameters listed in Table 2.2. The fitting performances of these five models are quantified using Squared-Error Sum (SES) measure and are compared in Table 2.3. The odd-order polynomial model (2.13) and the polysine model (2.14) are both analytic and much better fitted to the real data than the Cann model (2.2). This is illustrated in Figure 2.7 with sub-figures focusing on segments with significant differences where the data are rather harder to 61 Table 2.3: Approximation performance of five models (SES σ2e). Cann (2.2) 1.786 Model SES 0.963 0.533 0.346 0.032 Rapp (2.11) Polynomial (2.12) Polynomial (2.13) Polysine (2.14) fit. The better fitting performance is the closer to the data these curves ap- proach. With almost one order of magnitude better in SES than the rest, the polysine model's curve always coincide with all data points. The fitting performance of these models will be reflected in the nonlinearity simulation results discussed in section 2.4 bellow. 2.3.7. Other conventional HPA models Besides the AM-AM characteristic, updated envelope models for SSPAs at higher frequencies (of order GHz) and larger bandwidth (of order tens MHz) all consider the AM-PM conversion and generally better fit to the measured data than the previous models. However, it is not difficult to see that all models discussed below are not analytic or symmetric at r = 0 for most of the parameter sets and thus problem of (2.11) still exists. The AM-AM and AM-PM characteristics of these models are graphically illustrated in sub- figures 2.5(a) and 2.5(b) for comparison purpose. • Modified Saleh model: [72] is proposed for popular laterally diffused metal oxide semiconductor (LDMOS) HPAs, that are very common for the base station (BS) amplifiers of 2G, 3G and 4G mobile networks (in the L, S, 62 C bands). The AM-AM and AM-PM conversion functions are Fa(r) = αar√ 1 + βar3 , (2.17) Fp(r) = αp 3 √ 1 + r4 − εp, (2.18) where αa = 1.0536, βa = 0.086, αp = 0.161, and εp = 0.124 is a typical parameter set. • Modified Ghorbani model: [6] is suited for GaAs pHEMT (Gallium ar- senide pseudomorphic High-electron-mobility transistor) HPAs that op- erate at frequencies up to 28 GHz (K band, largely proposed for the 5G millimeter wave cells [96]) and are dominant in terms of production technologies and market shares compared to other power semiconductor technologies. This model assumes the following characteristics Fa(r) = α1r α2 + α3r α2+1 1 + α4rα2 , (2.19) Fp(r) = β1r β2 + β3r β2+1 1 + β4rβ2 , (2.20) where the model parameters are given by α1 = 7.851, α2 = 1.5388, α3 = −0.4511, α4 = 6.3531, β1 = 4.6388, β2 = 2.0949, β3 = −0.0325, β4 = 10.8217. • Modified Rapp model: [21] is introduced for GaAs pHEMT/CMOS (Com- plementary metal-oxide-semiconductor) HPA model operating at 60 GHz band, the new band for communication industry, with AM-AM function of (2.11) and AM-PM described as Fp(r) = αrq1( 1 + ( r β )q2) , (2.21) 63 where the parameter set are g = 16, Aos = 1.9, s = 1.1, α = −345, β = 0.17, q1 = q2 = 4. 2.4. Applications of HPA models in communication simulation This section describes the applications of envelope models investigated above for representing nonlinear HPAs in communication systems and anal- yses typical experiments with testing signals having discrete and continuous spectra to reveal the applicabilities and reasonableness of these models. 2.4.1. Representation of envelope models 0 0.1 0.2 0.3 0.4 0.5 0.6 0.7 0.8 0.9 1 −2.5 −2 −1.5 −1 −0.5 0 0.5 1 1.5 2 2.5 Figure 2.8: Two-tone waveform, f1 = 7 [Hz], f2 = 10 [Hz]. Consider the finding of IMPs in a two-tone test with a signal consisting of two equal-amplitude (A0/2) unmodulated sinusoid waveforms at frequencies f1 and f2 > f1. This (instantaneous) testing signal could be equivalently regarded as a double-sideband suppressed carrier AM of the form x inst (t) = 1 2 A0[sin(2pif1t) + sin(2pif2t)] = A0 cos(2pifmt) sin(2pifct), (2.22) where fm = 1 2 (f2−f1) is the modulating frequency, and fc = 12(f2+f1) is the (center) carrier frequency. Waveform (2.22) with f1 = 7 [Hz], f2 = 10 [Hz] is 64 illustrated in Figure 2.8. It is observed that the carrier fc (solid line) manifests inside the envelope and is the average of f1 and f2, while the envelope (dashed line) is the modulating signal at frequency fm. For representation convenience, with the 90o phase shifting of the modu- lating envelope component, (2.22) could be recast in the form of x inst (t) = A0 sin(2pifmt) sin(2pifct). (2.23) Therefore, its envelope form is x env (t) = A0 sin(2pifmt). (2.24) Since the envelope model requires non-negative input, thus, the sinusoid wave- form of (2.24) is decomposed into the polar form as x env (t) = r(t)ejφ(t) = A0| sin(2pifmt)|ejφ(t), (2.25) where r(t) = A0| sin(2pifmt)|, eφ(t) = 0, sin(2pifmt) > 0 pi, sin(2pifmt) < 0. In other words, the amplitude component r(t) is the full-wave-rectified sinu- soid, and the phase component φ(t) is the 180o square wave. Amplitude/ phase separator Envelope model ( )r t( )( ) j tr t e ( ) t ( )V t ( ) t ( ) t ( )( ) j tV t e Figure 2.9: Polar envelope model block diagram [52]. For simulating with envelope model in a general case of arbitrary r(t) and φ(t), the amplitude component r(t) is input to the model, while the 65 phase component φ(t) is bypassed as depicted in Figure 2.9. The distorted amplitude output V (t) is then combined with the phase part, resulting the output waveform for analysis. If AM-PM conversion is included, then the distorted phase Ψ(t) is added up to the input phase φ(t) resulting in output phase Φ(t), that finally is combined with V (t) in the polar form. 2.4.2. Simulation with two-tone testing signal −80 −70 −60 −50 −40 −30 −20 −10 −270 −240 −210 −180 −150 −120 −90 −60 −30 Input [dB] O ut pu t [dB ] Third−order IMPs Cann (2.2) Rapp (2.11) Polynomial (2.12) Polynomial (2.13) Polysine (2.14) (a) −80 −70 −60 −50 −40 −30 −20 −10 −300 −250 −200 −150 −100 −50 0 Input [dB] O ut pu t [dB ] Fifth−order IMPs Cann (2.2) Rapp (2.11) Polynomial (2.12) Polynomial (2.13) Polysine (2.14) (b) Figure 2.10: Third order (a) and fifth order (b) IMPs of five models in Figure 2.7. Simulation procedure is depicted in Figure 2.9 with the following parame- ters: simulation time 1 [s], sampling rate 1000 [Hz], input signal waveform as in Figure 2.8, five models with their corresponding characteristics depicted in Figure 2.7 are considered. Output signals will be used for IMPs analysis. The third- and fifth-order IMPs (IMP3/5) are respectively shown in sub- figures 2.10(a) and 2.10(b). As observed, Cann new model (2.2), odd order polynomial model (2.13) and polysine model (2.14) result in the required slope of 3 [dB/dB] and 5 [dB/dB] correspondingly for IMP3 and IMP5 as expected [38, 64]. With almost the same structure as (2.13), however, the full order polynomial model (2.12) fails in simulating the odd IMPs, revealing 66 the problem as found by Litva in [62] for Cann old model (2.1). So does the Rapp model. In these cases, full order polynomial model results in slope of 2 [dB/dB] for both IMP3 and IMP5, while this quantity is 9 [dB/dB] for Rapp model. They are obviously irrelevant and quite different. Surely, those are the differences in non-analyticities of these models that create the results. It can be seen that IMPs are much smaller in magnitude than the funda- mental component (input) signal. Further, for three analytic models, there are different constant gaps between IMPs created. Obviously, smaller error in fitting approximation should result in better performance of simulation, par- ticularly for small-value components. Thus, Cann new model (2.2) produces less confident results than what created by odd-order polynomial model (2.13) and especially by polysine model (2.14). 0 0.25 0.5 0.75 1 1.25 1.5 1.75 2 2.25 0 0.5 1 1.5 2 2.5 x 10 4 (a) 0 0.2 0.4 0.6 0.8 1 1.2 1.4 1.6 1.8 0 500 1000 1500 2000 2500 3000 (b) Figure 2.11: Amplitude histograms of two-tone (a) and 1+7-APSK (b) testing sig- nals. Reconsider the signal processing depicted in Figure 2.9, it is recognized that the amplitude/phase separator indirectly yields the modulus operation, causing the former problem. Thus, to receive reasonable results for the two- tone test, the envelope model should be analytic at r = 0, as the same as 67 what found by Loyka [65] for the instantaneous model. To clearly see the defect of Rapp model (2.11) and polynomial model (2.12) under the effect of the signal amplitude distribution to the IMPs created, consider the amplitude histogram of this two-tone testing signal illustrated in sub-figure 2.11(a). It is undoubtedly inferred that, for non-analytic mod- els, the very high concentration of signal amplitude around r = 0 results in irrelevant model outputs, of which some or many frequency components disappeared. This finally yields the failure in calculating IMPs as illustrated in sub-figures 2.10(a) and 2.10(b). 2.4.3. Simulation with continuous-spect
File đính kèm:
luan_an_nonlinear_distortions_and_countermeasures_for_perfor.pdf
Thong tin ve LA dua len mang_Thanh.doc
TomTatLA_Thanh.pdf