Luận án Person re-identification in a surveillance camera network
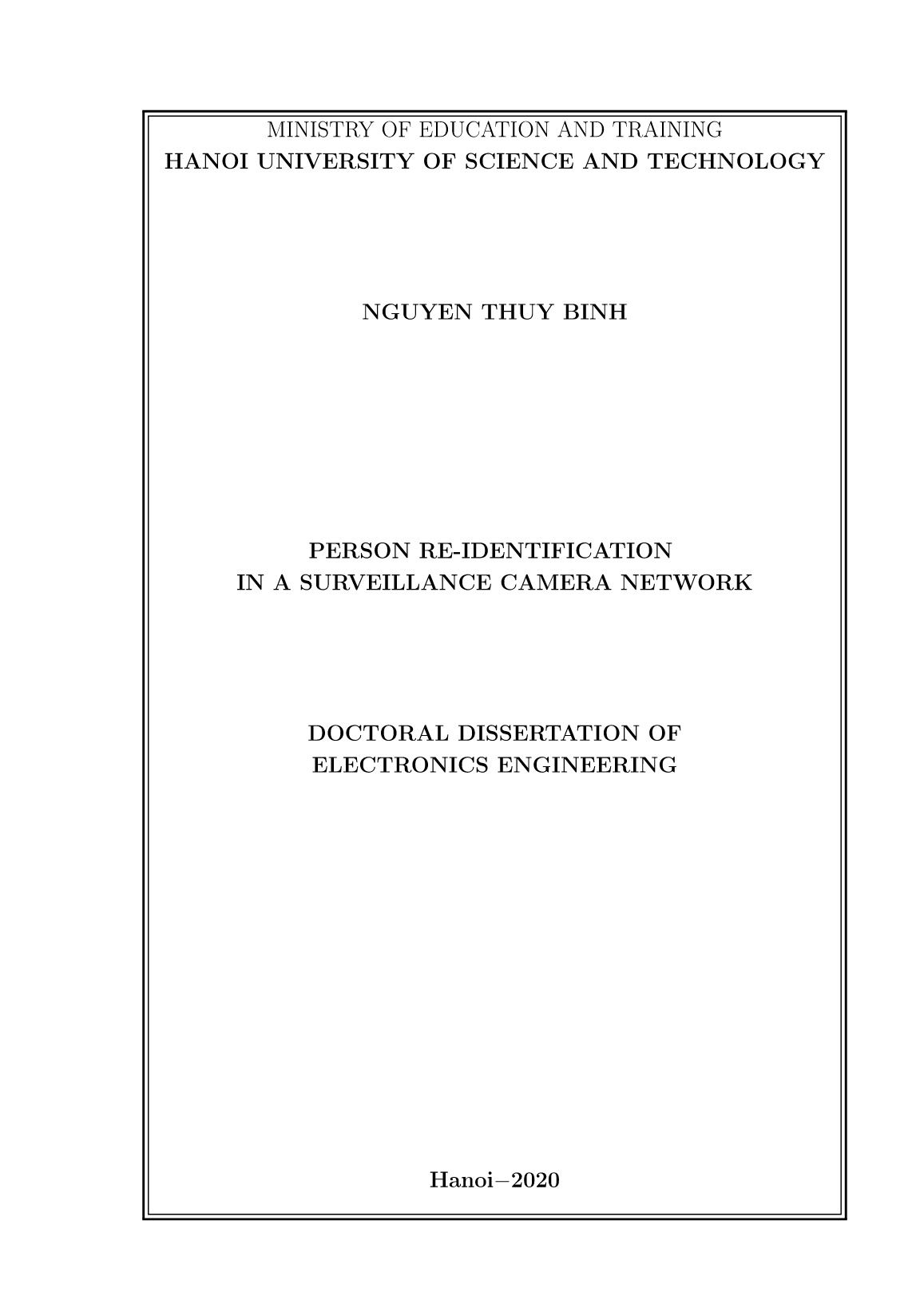
Trang 1
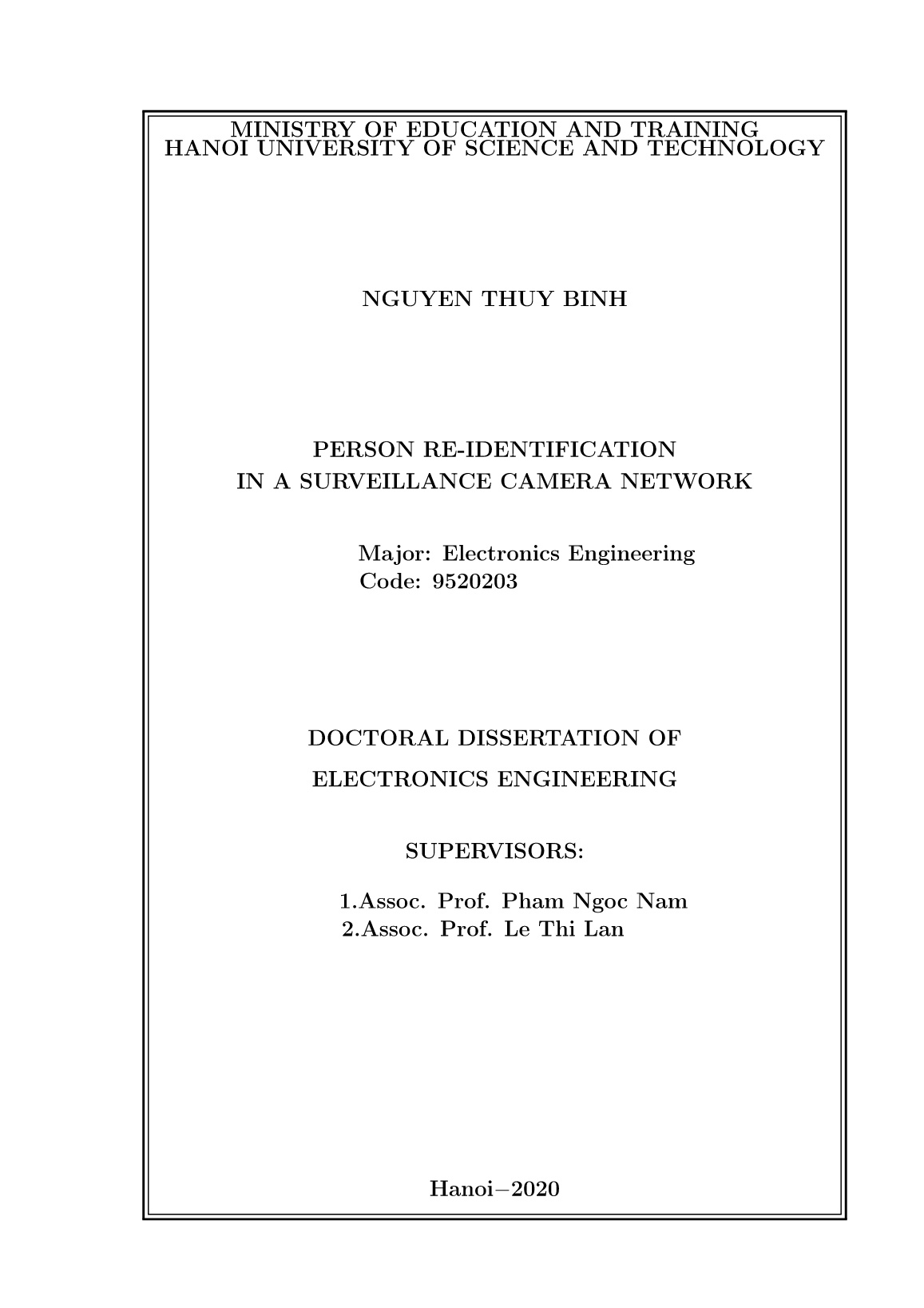
Trang 2
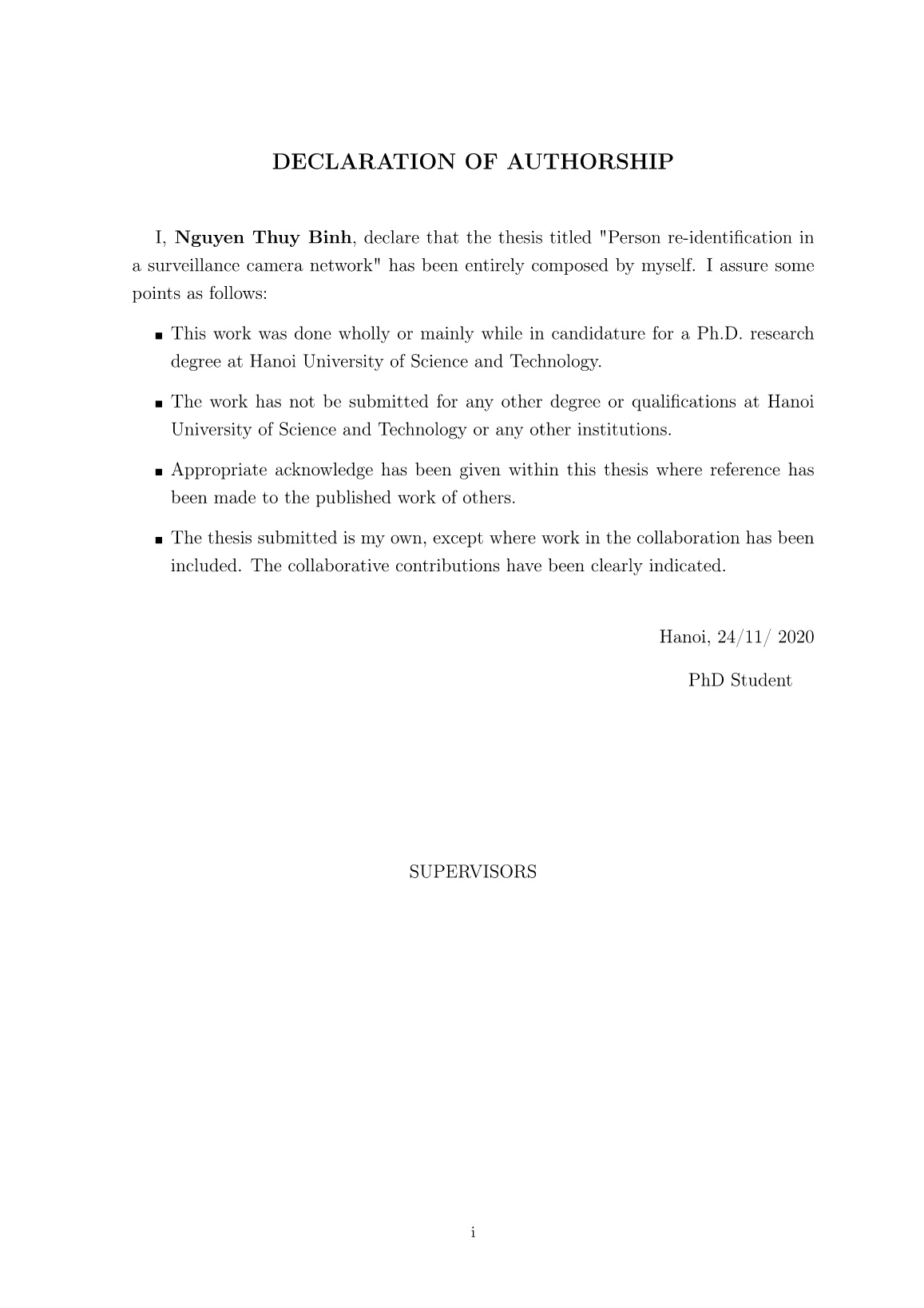
Trang 3
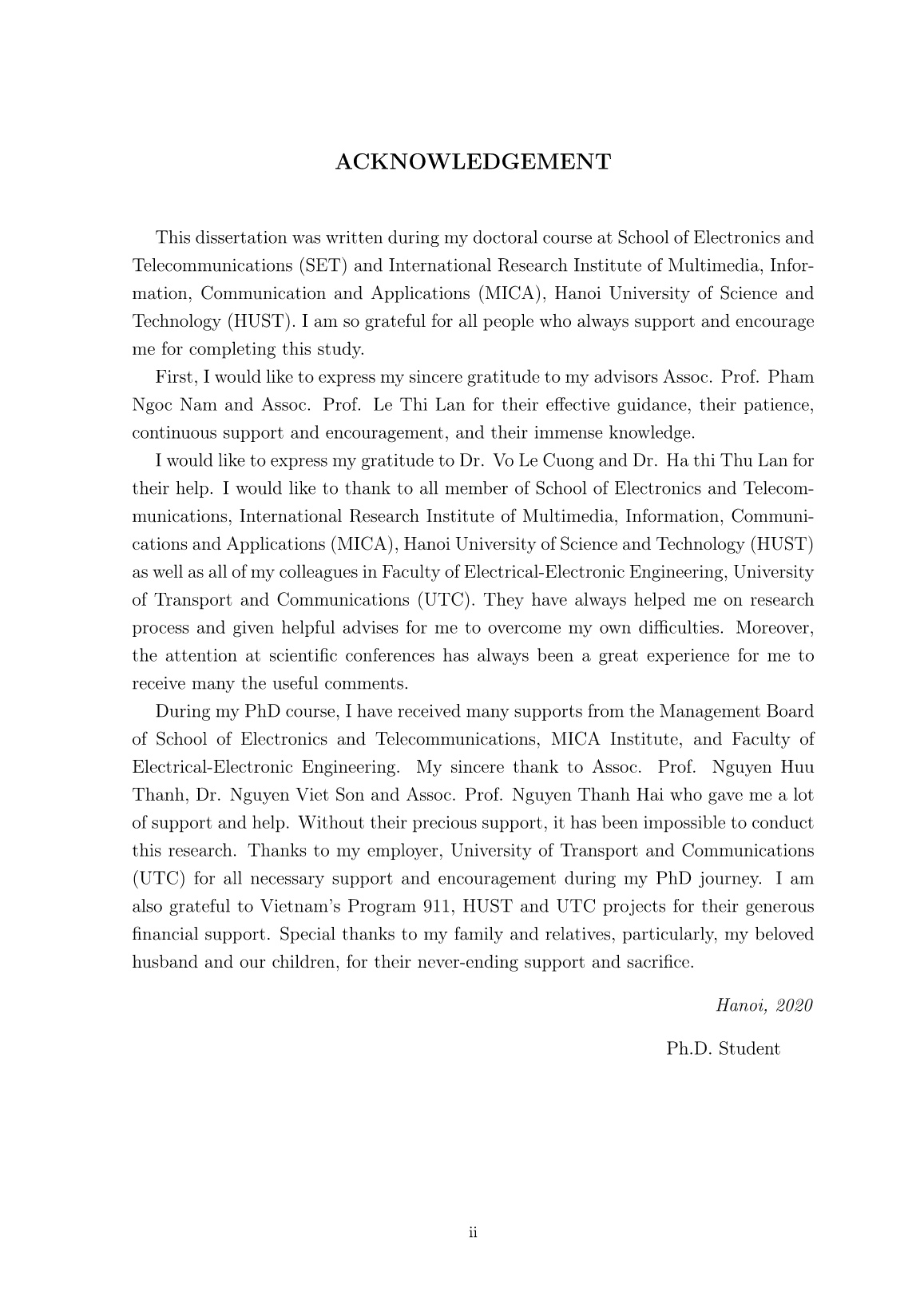
Trang 4
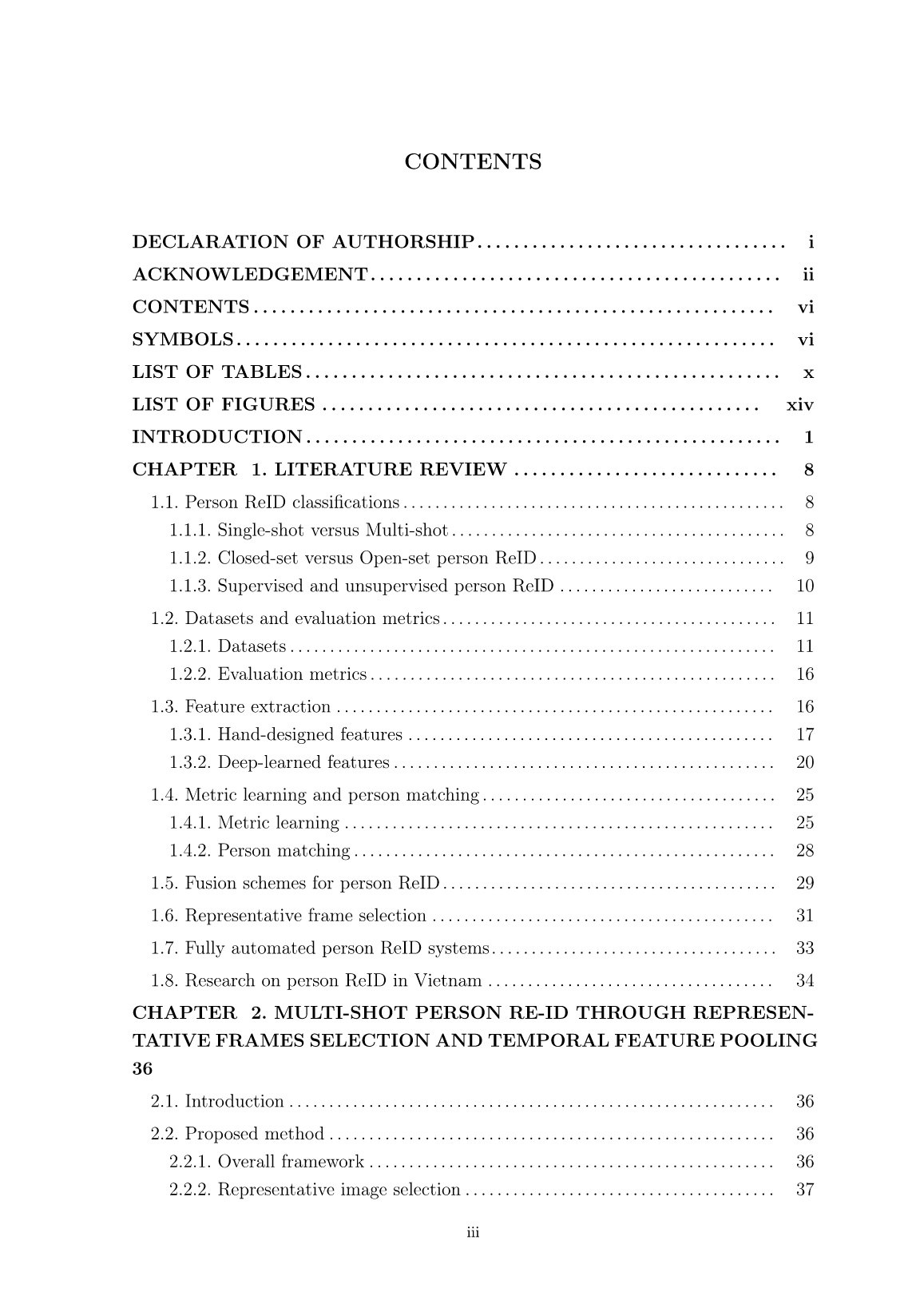
Trang 5
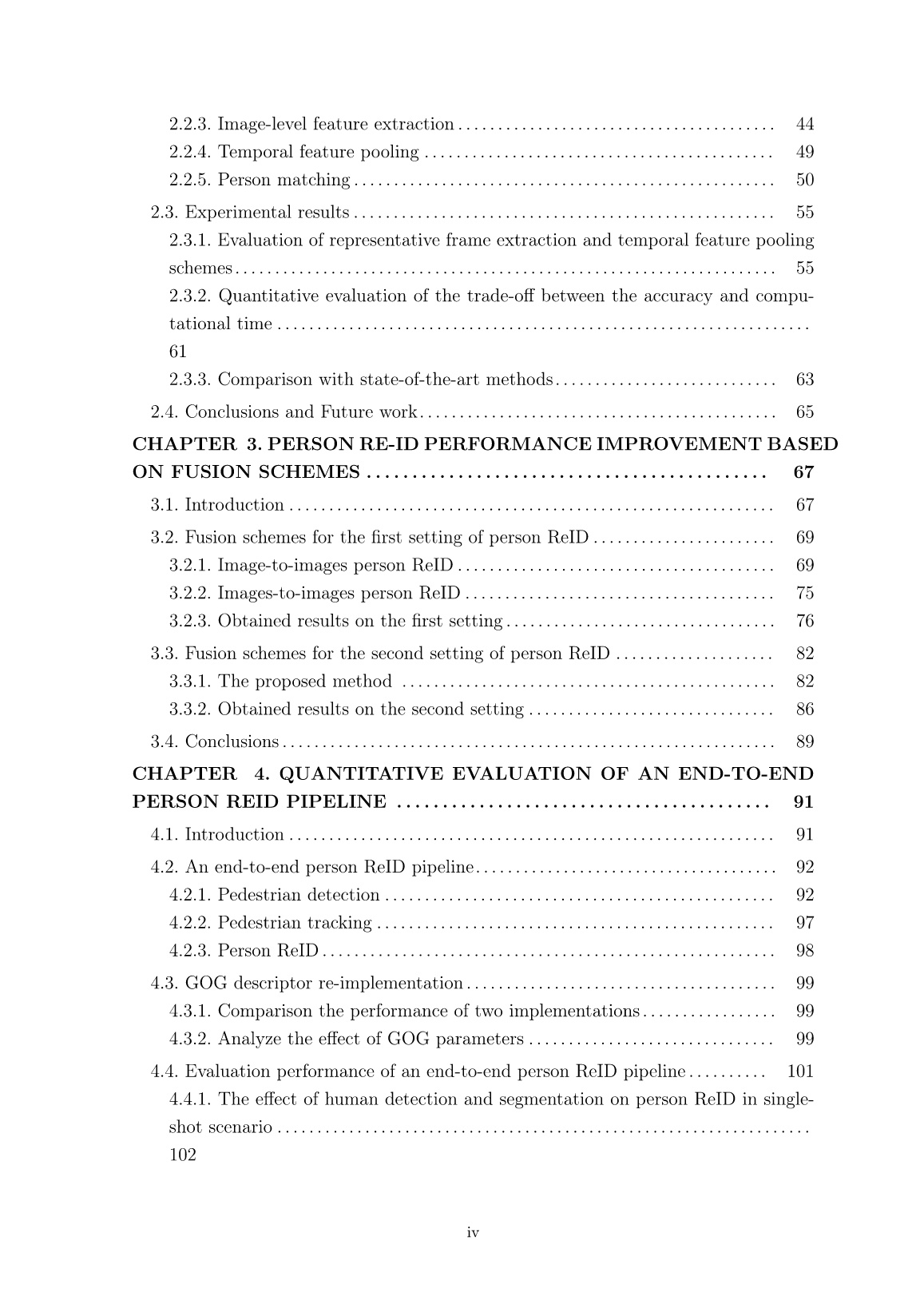
Trang 6
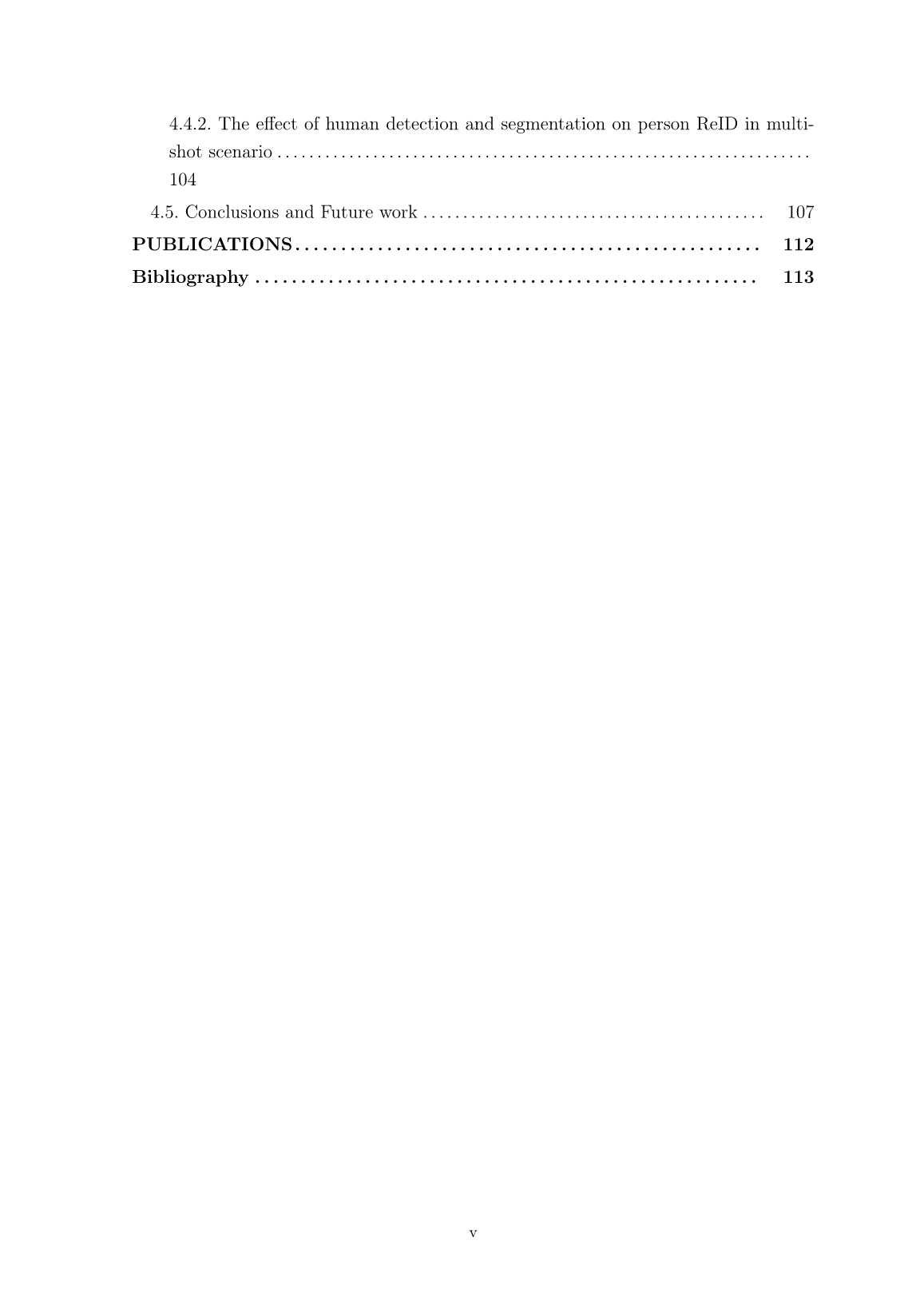
Trang 7
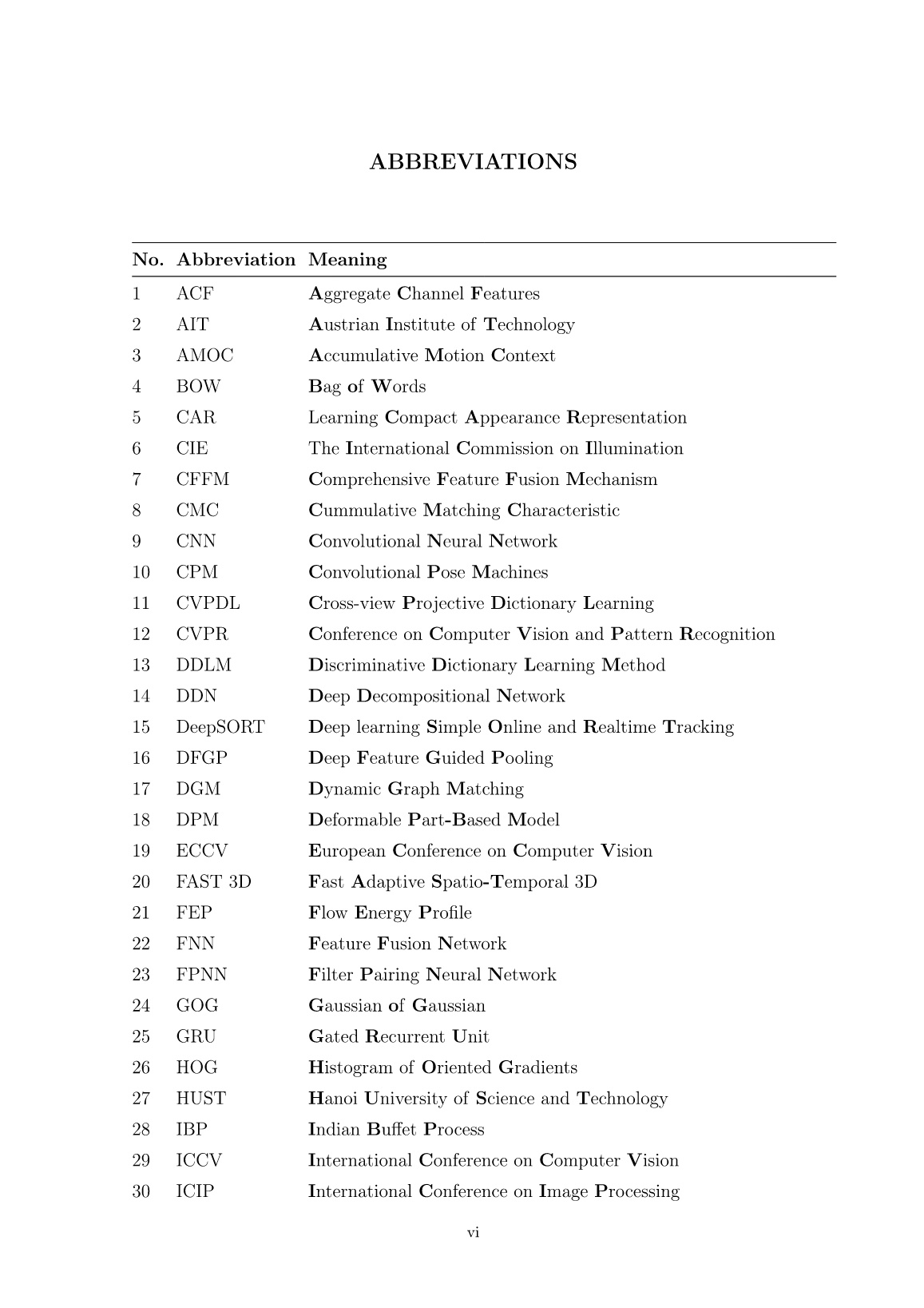
Trang 8
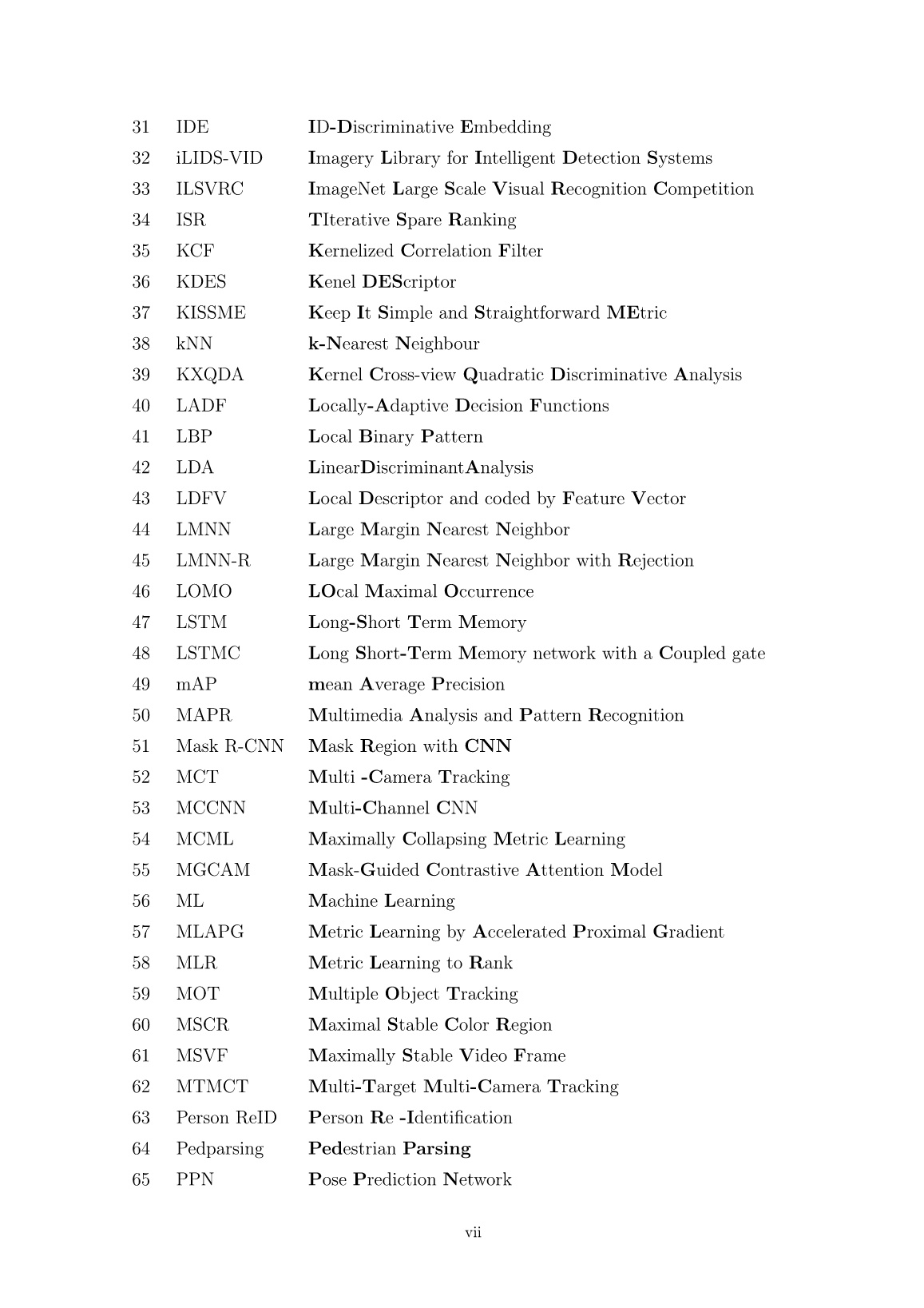
Trang 9
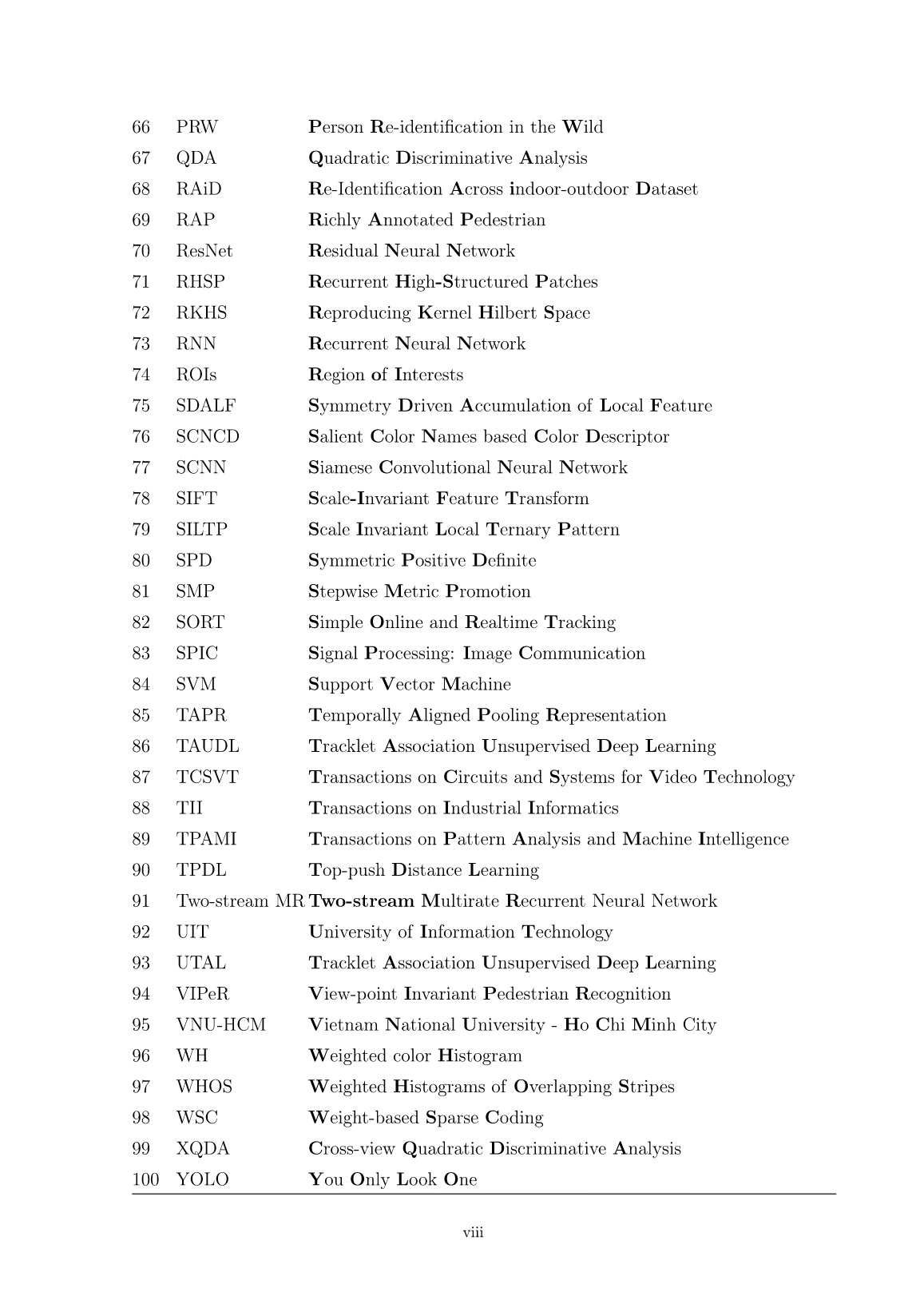
Trang 10
Tải về để xem bản đầy đủ
Bạn đang xem 10 trang mẫu của tài liệu "Luận án Person re-identification in a surveillance camera network", để tải tài liệu gốc về máy hãy click vào nút Download ở trên.
Tóm tắt nội dung tài liệu: Luận án Person re-identification in a surveillance camera network
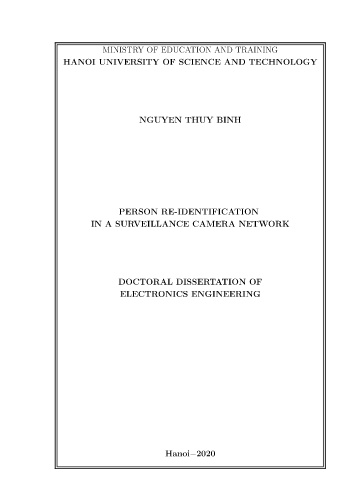
ed when feature vectors are extracted on HSV color space. In comparison with the other color spaces, the matching rates at rank-1 when working on HSV color space are the most strongly increased by 3.91% and 4.35% compared to the worst results in case of using four key frames and walking cycle, respectively. However, this conclusion is not true with obtained results on iLIDS-VID dataset. By 57 Table 2.4: The matching rates (%) when applying different pooling methods on different color spaces in case of using four key frames on iLIDS-VID dataset. The two best results for each case are in bold. Color spaces Rank Methods AVG-AVG AVG-MAX AVG-MIN MAX-AVG MAX-MAX MAX-MIN MIN-AVG MIN-MAX MIN-MIN RGB r=1 38.13 32.93 33.49 33.12 34.63 27.59 32.83 27.35 34.89 r=5 66.71 59.91 60.41 60.07 62.72 52.52 59.64 52.72 62.92 r=10 78.61 72.52 72.98 72.54 75.03 65.52 72.00 65.67 75.11 r=20 88.60 84.37 84.35 84.29 86.59 77.99 83.87 78.98 86.07 Lab r=1 38.21 33.24 32.30 32.94 35.26 26.91 32.83 28.18 34.52 r=5 66.43 60.47 59.29 59.77 63.66 51.88 59.34 53.06 61.68 r=10 78.70 72.91 72.13 72.54 75.83 64.52 71.75 65.83 74.29 r=20 88.69 84.67 83.95 84.33 87.14 77.95 83.87 78.92 85.88 HSV r=1 34.75 31.20 29.34 30.21 32.29 24.25 31.40 26.60 31.85 r=5 62.52 58.73 56.90 57.57 60.87 50.05 58.76 52.34 59.67 r=10 75.38 71.41 69.50 70.65 73.69 63.30 71.19 65.66 72.43 r=20 86.31 83.05 82.19 83.01 85.19 77.28 83.42 78.59 84.33 nRnG r=1 37.77 32.30 32.11 32.75 34.41 26.77 33.89 27.78 35.16 r=5 65.74 59.02 58.67 59.30 62.17 51.34 60.05 52.93 62.75 r=10 77.47 71.49 71.06 71.46 74.38 63.75 71.85 64.90 74.57 r=20 87.48 82.87 82.87 83.15 85.34 76.23 83.54 77.55 85.67 Fusion r=1 41.09 36.63 35.99 36.11 38.31 30.13 36.23 31.35 38.20 r=5 69.51 64.49 63.41 63.68 66.94 56.06 63.52 57.59 66.28 r=10 80.94 76.44 75.91 75.58 78.89 68.46 75.56 70.01 78.11 r=20 90.43 86.79 86.69 86.27 88.82 81.21 86.43 82.51 88.38 Table 2.5: The matching rates (%) when applying different pooling methods on different color spaces in case of using frames within a walking cycle on iLIDS-VID dataset. The two best results for each case are in bold. Color spaces Rank Methods AVG-AVG AVG-MAX AVG-MIN MAX-AVG MAX-MAX MAX-MIN MIN-AVG MIN-MAX MIN-MIN RGB r=1 41.09 32.98 33.37 34.38 37.83 26.93 33.73 27.37 38.03 r=5 68.75 58.95 58.63 60.01 66.15 50.75 59.51 51.63 65.53 r=10 80.21 70.71 70.27 71.87 77.54 62.41 71.57 64.55 77.05 r=20 89.39 82.15 81.73 83.05 87.93 75.27 82.57 77.27 87.50 Lab r=1 41.20 34.33 32.01 34.09 38.90 26.43 33.85 28.39 37.19 r=5 69.27 59.35 57.02 59.41 66.51 49.53 59.23 52.55 64.47 r=10 80.21 71.26 69.08 71.60 78.49 61.58 71.32 64.59 76.21 r=20 89.55 82.47 80.91 83.26 88.33 74.50 82.75 77.37 87.15 HSV r=1 37.01 30.93 28.82 31.26 35.70 23.36 31.88 25.75 34.64 r=5 64.73 57.39 54.47 58.08 63.57 47.66 59.43 50.85 62.30 r=10 76.19 69.41 67.07 70.55 75.80 60.64 71.65 63.95 74.59 r=20 86.80 80.99 79.37 82.51 86.61 74.51 82.89 76.55 85.49 nRnG r=1 41.51 32.90 31.00 34.33 38.36 26.02 34.86 28.21 38.89 r=5 68.75 58.84 56.23 59.33 66.03 48.75 60.56 52.55 65.48 r=10 79.55 70.15 68.14 71.36 77.24 60.82 72.00 64.61 77.28 r=20 88.91 81.50 79.23 82.35 87.55 73.33 82.68 77.03 87.33 Fusion r=1 44.14 36.89 35.49 37.19 41.37 29.69 37.29 31.68 41.19 r=5 71.71 63.18 61.15 63.52 69.77 54.00 63.87 56.74 68.64 r=10 82.40 74.35 72.97 75.10 80.62 66.51 75.03 68.87 79.97 r=20 90.63 84.82 84.10 85.49 89.71 78.53 85.45 80.71 89.19 58 Table 2.6: The matching rates (%) when applying different pooling methods on different color spaces in case of using all frames on iLIDS-VID dataset. The two best results for each case are in bold. Color spaces Rank Methods AVG-AVG AVG-MAX AVG-MIN MAX-AVG MAX-MAX MAX-MIN MIN-AVG MIN-MAX MIN-MIN RGB r=1 58.20 47.93 48.87 46.80 50.87 23.80 45.47 24.73 52.80 r=5 80.13 71.93 70.73 71.13 77.73 46.40 72.07 46.20 75.80 r=10 87.47 82.07 79.40 81.13 85.73 57.73 82.13 57.73 83.93 r=20 92.27 89.80 87.20 89.47 91.47 69.00 89.27 73.73 89.80 Lab r=1 58.80 51.27 46.93 45.87 54.47 22.33 46.33 26.40 50.00 r=5 81.00 75.13 71.67 73.07 79.73 42.07 71.87 47.53 76.00 r=10 87.47 83.93 80.00 82.13 87.60 53.73 81.73 61.13 84.07 r=20 92.93 90.87 88.60 89.73 92.13 66.73 88.53 73.80 89.53 HSV r=1 54.67 46.40 44.27 43.87 50.47 21.00 44.27 19.47 49.53 r=5 78.73 72.73 71.20 70.60 77.87 46.40 71.00 44.13 76.80 r=10 84.93 80.73 80.80 80.87 85.93 60.13 81.53 56.80 84.60 r=20 92.07 89.47 88.73 88.87 92.13 72.73 88.73 69.80 90.47 nRnG r=1 58.93 50.13 49.13 46.13 50.87 25.67 47.00 28.53 50.80 r=5 79.93 73.33 72.27 72.40 76.13 46.13 72.33 51.53 75.87 r=10 86.73 82.53 79.73 81.40 84.73 58.73 81.80 62.60 84.60 r=20 92.40 89.73 86.93 89.53 90.80 69.67 89.33 73.07 89.93 Fusion r=1 70.13 55.40 52.93 49.27 56.33 26.27 49.93 29.13 56.20 r=5 92.73 77.47 77.27 75.33 81.47 49.20 76.40 51.60 80.40 r=10 97.33 86.07 84.67 84.67 88.40 62.13 84.33 63.53 86.80 r=20 99.07 91.53 90.80 91.67 93.07 74.20 91.13 76.60 91.87 5 10 15 20 40 50 60 70 80 90 100 Ma tch ing rat es (%) Rank 90.56% PRID_all frames 79.10% PRID_walking cycle 77.19% PRID_4 key frames (a) 5 10 15 20 30 40 50 60 70 80 90 100 M a t c h i n g r a t e s ( % ) Rank 41.09% iLIDS_4 key frames 44.14% iLIDS_walking cycle 70.13% iLIDS_all frames (b) Figure 2.14: Evaluation the performance of GOG features on a) PRID 2011 dataset and b) iLIDS-VID dataset with three different representative frame selection scheme. 59 contrast, for iLIDS-VID dataset, the matching rates at rank-1 when working on HSV color space are the worst results. The matching rates at rank-1 in case of working on HSV color space are the most drastically decreased by 4.15% and 4.55% compared to the best results. From these analysis, we can realize that each kind of color models has its own effectiveness depending on evaluated dataset. And, by combining features on different color space will bring a higher performance for this problem. From Tables 2.1-2.3, by applying the fusion strategy, the matching rates at rank-1 on PRID-2011 are improved by 6.99%, 6.62%, and 14.50% compared to the worst ones in case of using four key frames, walking cycle, and all frames, respectively. For iLIDS-VID, the highest improvements are 6.65%, 7.13%, and 7.47% when using four key frames, walking cycle, and all frames, respectively. These results show more clearly the effectiveness of the fusion scheme in achieving a better performance for person ReID problem. 5 10 15 20 30 40 50 60 70 80 90 100 CMC curves on PRID-2011 Ma tch ing rat es (%) Rank 77.19% 4 key frames 76.53% 4 random frames (a) 5 10 15 20 30 40 50 60 70 80 90 100 CMC curves on iLIDS-VID dataset Ma tch ing rat es (%) Rank 41.01% 4 key frames 39.03% 4 random frames (b) Figure 2.15: Matching rates when selecting 4 key frames or 4 random frames for person representation in a) PRID-2011 and iLDIS-VID In order to prove that four key frames are the best choice for balance between ReID accuracy and computation time, an extra scenario is performed. In which, four key frames are chosen randomly in a walking cycle. Noted that in this experiments, average pooling is still used for generating the final signature of each person and the results are obtained in case of combining all feature vectors in four color spaces (RGB, Lab, HSV, nRnG). Figure 2.15 shows the matching rates in two considered scenarios including four key frames and four random frames in both evaluated datasets PRID-2011 and iLIDS-VID. By observing these obtained results, CMC curves corresponding to four random frames are located at lower position compared those corresponding to four key frames in both PRID-2011 and iLIDS-VID datasets. This means that by choosing four key frames, the proposed framework can achieve a higher performance and it is also the reason why four key frames are considered in this chapter. 60 Table 2.7 shows the matching rates in several important ranks (1, 5, 10, 20) in the two above considered scnarios. Additionally, these obtained results are also compared to the reported one in the study of Nguyen et al. [58], in which one frame of each person in PRID-2011 is chosen randomly and multi-shot problem is turned into single- shot one. It is obvious that the matching rates in case of using four key frames are much higher than those in case of choosing only one frame for each person. Table 2.7: Matching rates (%) in several important ranks when using four key frames, four random frames, and one random frame in PRID-2011 and iLIDS-VID datasets. Dataset PRID-2011 iLIDS-VID Methods Rank=1 Rank=5 Rank=10 Rank=20 Rank=1 Rank=5 Rank=10 Rank=20 Four Key Frames 77.19 94.70 97.93 99.37 41.09 69.51 80.94 90.43 Four Random Frames 76.53 93.83 97.47 99.29 39.03 67.09 78.27 88.18 One Random Frame [58] 53.60 79.76 88.93 95.79 - - - - 2.3.2. Quantitative evaluation of the trade-off between the ac- curacy and computational time As mentioned in the previous section, using all frames of a person achieves the best ReID performance. However, this scheme requires high computational time and memory storage. In this section, a quantitative evaluation of these schemes is indicated to point out the advantages and disadvantages of the proposed representative frame selection schemes. Table 2.8 shows the comparison of the three schemes in terms of person ReID accuracy, computational time, and memory requirement on PRID 2011 dataset. Table 2.8: Comparison of the three representative frame selection schemes in term of accuracy at rank-1, computational time, and memory requirement on PRID 2011 dataset. Methods Accuracy at rank-1 Computational time for each person (s) Memory Frame selection Feature extraction Feature pooling Person matching Total time Four key frames 77.19 7.500 3.960 0.024 0.004 11.488 96 KB Walking cycle 79.10 7.500 12.868 0.084 0.004 20.452 312 KB All frames 90.56 0.000 98.988 1.931 0.004 100.919 2,400 KB The computational time for each query person includes the computational time for representative frame selection, feature extraction, feature pooling, and person match- ing. It is worth to note that the computational time for person matching depends on the size of the dataset (e.g. the number of individuals). The values reported in Table 2.8 are computed for a random split on PRID 2011 dataset. This dataset contains 178 pedestrians in which 89 individuals are used for the training phase and the rest of dataset is used for the testing phase. The average number of images for each person on both camera A and B is about 100, and each walking cycle has approximately 13 61 (a) 6 (b) Figure 2.16: The distribution of frames for each person in PRID 2011 dataset with a) camera A view and b) camera B view. images on average. The experiments are conducted on Computer Intel(R) Core(TM) i5-4440 CPU @ 3.10GHz, 16GB RAM. As shown in this Table, using all frames does not require frame selection, therefore the computational time for frame selection of this scheme is zero. These two other schemes spend 7.5s for extracting walking cycles and selecting four-key-frames. Concerning computational time for feature extraction step, for each image with the resolution 128× 64, the implementation takes about 0.99s to extract feature vectors on all color spaces (RGB/Lab/HSV/nRnG) and concatenate them together. The time for feature extraction are 3.96s, 12.87s, and 98.99s in case of using four key frames, a walking cycle, and all frames, respectively. The computational time spent for pooling data is 0.024s, 0.084s, and 1.931s corre- sponding to the cases of four key frames, a walking cycle, and all frames, respectively. In person matching step, training phase is performed off-line so the execution time is calculated for the testing phase. After data pooling layer, each individual is represented by a feature vector, so the computational time in this step is considered as constant value for all different cases, with 0.004s. The total computational time for each query person is 11.488s, 20.452s, and 100.919s for four key frames, one walking cycle, and all frames, respectively. Concerning the memory requirement, as an image of 128 × 64 pixels with a 24-bit color depth takes 24KB (128× 64× 24 = 196, 608 bits =24 KB), the required memory for four key frames, one walking cycle and all frames schemes are 96KB (24×4), 312KB (24× 13), and 2,400KB (24× 100 = 2, 400) respectively. It can be seen from the experimental results that, using all frames in a cycle walking help to increase approximately 2% of matching rate at rank-1, while the computational time is nearly twice in comparison with four key frames case. Among three schemes, 62 using all frames achieves impressive results (the accuracy at rank-1 is 90.56%), however, it requires higher computational time (8.79 times higher than that of four key frames scheme) and memory (25 times higher than that of four key frames scheme). To see in detail the requirement of this scheme, the distribution of the frames for each person in PRID 2011 dataset (see Fig.2.16) is computed. As seen from the Figure, in PRID 2011 dataset, there are 78.09% and 41.01% of total people having more than 100 frames in camera A and camera B, respectively. This means that in surveillance scenarios, the number of available frames for a person is high in majority cases. There- fore, using all of these frames costs computational time and occupied memory while it’s performance is superior than thoses of four key frames and one walking cycle at rank-1. For these higher ranks, the difference between these accuracies is not significant. For example: the accuracies at rank-5 of using four key frames, one walking cycle, and all frames are 94.70%, 94.99%, and 97.98% respectively while those at rank-10 are 97.93%, 97.92%, and 99.55%. The evaluation results allow us to suggest the recommendation for the use of these representative frame selection schemes. If the dataset of working application is chal- lenging and the application requires relevant results returned in few first ranks, using all frames scheme for person representation is recommended. This scheme is also a suitable choice when the powerful computation resources are available. Otherwise, four key frames and one cycle walking schemes would be considered. 2.3.3. Comparison with state-of-the-art methods This section aims to compare the proposed method with the state of the art works for person ReID in two benchmark datasets of PRID 2011 and iLIDS-VID. The methods are arranged into two groups: the unsupervised learning based methods and supervised learning based methods. This study belongs to the supervised learning group. The comparisons are shown in Table 2.9 with two best results are in bold. The comparison shows three observations. Firstly, in comparison with unsupervised methods, the proposed method outper- forms almost unsupervised learning-based methods on both PRID 2011 and iLIDS-VID datasets. Remarkably, the results from PRID 2011 with three cases: four key frames, all frames in a walking cycle and all frames are 77.2%, 79.1% and 90.5% at rank 1, re- spectively. They are much higher than the state-of-the-art proposals of TAUD (49.4%) [133] and UTAL (54.7%) [134]. This also happens for iLIDS-VID, with 41.1%, 44.1% and 70.1% of the proposed method compared to 26.7% [133] and 35.1% [134]. Among the considered unsupervised methods, the method SMP [24] that uses each training tracklet as a query and performs retrieval in the cross-camera training set provides a little higher result than the proposed method at a walking cycle case for PRID (80.9% compared to 79.1%) but lower for iLIDS-VID (41.7% compared to 44.1%) at rank-1. 63 However, for the case of all frames, matching rates at rank-1 of the proposed methods stand at higher numbers for both of these datasets in comparison with SMP method (90.5% against 80.9% and 61.7% against 41.7%). The performance gains by the pro- posed method are due to the robustness of the feature used for person representation and the metric learning XQDA. Secondly, in comparison with the methods in the same group (supervised learning- based methods), the proposed method outperforms all of 11 state-of-the art methods at the rank-1 for PRID 2011 dataset. Interestingly, the proposed method brings a significant ReID accuracy in comparison with the deep learning-based methods with PRID 2011 dataset. The rank-1 margins by the proposed method is 11.8% (90.5%- 78.7%) and 20.5% (90.5%-70.0%) more than the methods using deep recurrent neural network [135], [57], respectively. When working with the dataset iLIDS-VID that is suffered from strong occlusions, the proposed method still obtains good results for rank- 1. However, the performance of the proposed method is inferior than two methods in the list [135], [136]. Among the state-of-the-art methods, the method in [136] obtained impressive results for both PRID 2011 and iLIDS-VID. However, this method requires a lot of computation as they exploited both appearance and motion features extracted through a two-stream convolutional network architecture. Finally, a close-up comparison with state-of-the-art methods [102, 100, 101] that are related closely to the proposed work is given. These works all extracted walking cycles for the representative frame selection before feature extraction step based on motion energy. For PRID 2011 dataset, it can be seen the outperforming results of the proposed framework compared to HOG3D [102] and STFV3D [100]. The matching rate at rank-1 of CAR [101] is superior than those of the proposed framework, nevertheless, at higher ranks (e.g. rank-5, rank-20), the proposed method achieves better performance. For iLIDS-VID dataset, the proposed method still outperforms the method HOG3D [102] and obtains competitive results in comparison with the method STFV3D [100]. In order to prove more clearly the effectiveness of the proposed framework in this Chapter compared with related existing studies, we are going to analyze and discuss on three studies reported in [103, 104, 105]. All of these studies relate to representative frames selection based on a clustering algorithm. In [103], representative frames are chosen via computing the similarity between two consecutive images via Histogram of Oriented Gradient descriptor extracted on each frame. And then, a curve based on consecutive image similarities is plotted, the local minimal of the curve corresponding to a high variation in the appearance are found. Via these minimal points, the trajectory is divided into several segmentation. Each part models a posture variation. The key frame of each cluster is chosen by taking the centered image. It is clear that representative frames are chosen based on the similarity between two consecutive frames which strongly depends on human pose when he/she remove in a camera network. 64 Additionally, the number of images in each fragment are different. These will be shown more clearly in different field of camera views. Inversely, in the proposed framework in this Chapter, motion energy is computing only on the lower human body which more stable and robust than computing HOG descriptor on all human body in [103]. In [104, 105], the authors proposed to exploit Covariance descriptor for image representation and Mean-shift algorithm for clustering person images into several group. However, several groups which have the number of images smaller than average number of images in all clusters are removed. Representative frames are chosen as frames that have the minimum distance to the center of each cluster. The research in [105] is an extended version of [104] in which three descriptors including HOG, Covariance, and multi-
File đính kèm:
luan_an_person_re_identification_in_a_surveillance_camera_ne.pdf
2.Abstract_Vietnamese.pdf